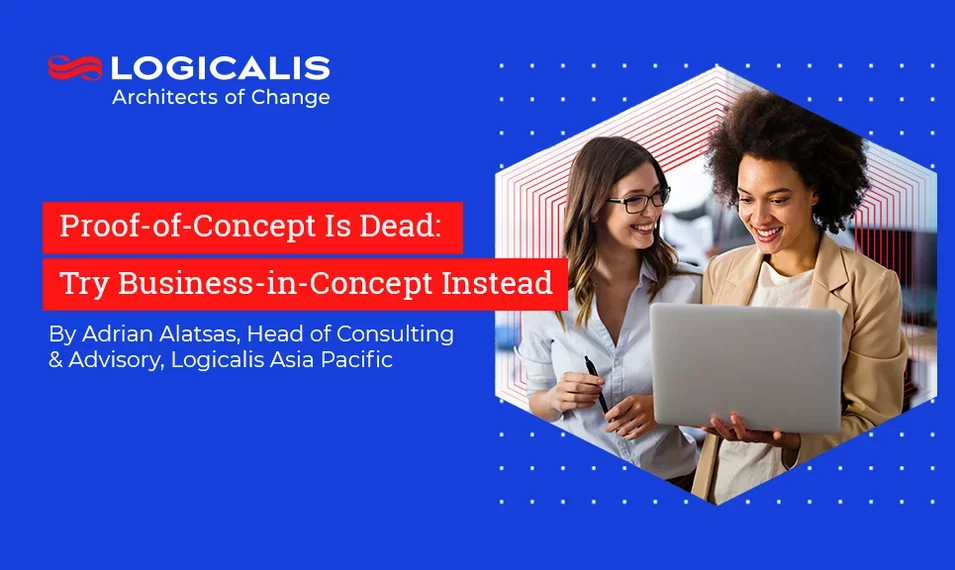
Australia, Apr 3, 2025
For years, the Proof-of-Concept (PoC) was seen as the safe way to test new technology.
- Low investment
- Clear scope
- Fast timelines
- Technical validation
It made perfect sense in the traditional IT project lifecycle.
But when it comes to AI, the classic PoC approach is fundamentally broken.
Here’s why: AI doesn’t just need to work — it needs to create value. And value doesn’t come from technical validation. It comes from solving real business problems in real business environments.
The Problem with the Classic AI PoC
In many AI PoCs, the same pattern plays out:
- A model is built in a test environment
- Synthetic or small sample data is used
- The use case is selected for simplicity, not strategic importance
- The business is kept at arm’s length to avoid “slowing it down”
What happens next?
- The PoC technically works — but has no path to operationalisation
- The use case has no meaningful value story
- The business wasn’t engaged — so no one owns it
- The initiative dies in the “value gap” between IT and the organisation
It’s no wonder so many AI pilots never make it past the pilot phase.
AI Needs to Be Grounded in Business from Day One
AI is not a tool you plug in. It’s a capability you build. That means the success of an AI initiative depends on:
- Having a real business sponsor
- Aligning the initiative with an actual pain point or opportunity
- Using real, messy data — not curated samples
- Understanding the downstream process and decision implications
- Measuring success through business outcomes, not technical performance
In other words, AI needs to be business-in-concept, not just proof-of-concept.
What Business-in-Concept Looks Like
A business-in-concept approach flips the traditional PoC model on its head.
Instead of asking:
- “Can the model work?”
You ask:
- “Can this improve how we operate, decide, or compete?”
And instead of building tech in a vacuum, you:
- Engage business leaders early
- Define the value hypothesis clearly
- Map the user journey or workflow the AI will impact
- Build around a real-world process with real-world data
- Ensure it’s embedded into how decisions are made
This approach makes AI real — fast. It creates momentum. And it starts to build the internal capability and change muscle your business actually needs.
Avoiding the Governance Trap
Business-in-concept also forces you to confront your information landscape early:
- Do you trust your data enough to make AI-informed decisions?
- Are your governance policies clear enough to allow responsible use?
- Can your team actually act on what the AI recommends?
Instead of hiding these issues under the hood of a “controlled test,” you address them up front — and make progress where it counts.
Why This Matters Now
The organisations making real progress with AI aren’t doing more pilots. They’re doing better, more integrated experiments.
- Experiments that are tied to real outcomes
- Experiments that elevate internal knowledge
- Experiments that drive cross-functional collaboration
- Experiments that uncover and fix foundational blockers
Because at this stage in the AI journey, the goal isn’t to test the tech. The goal is to build the business muscle to scale it.
Related Insights
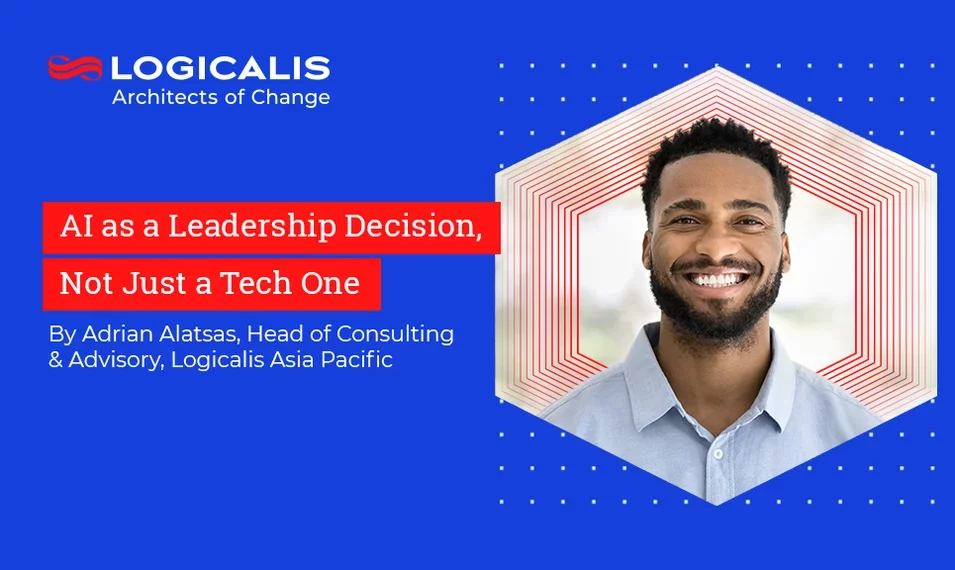
Australia , Apr 3, 2025
AI as a Leadership Decision, Not Just a Tech One
By Adrian Alatsas, Head of Consulting & Advisory, Logicalis Asia Pacific
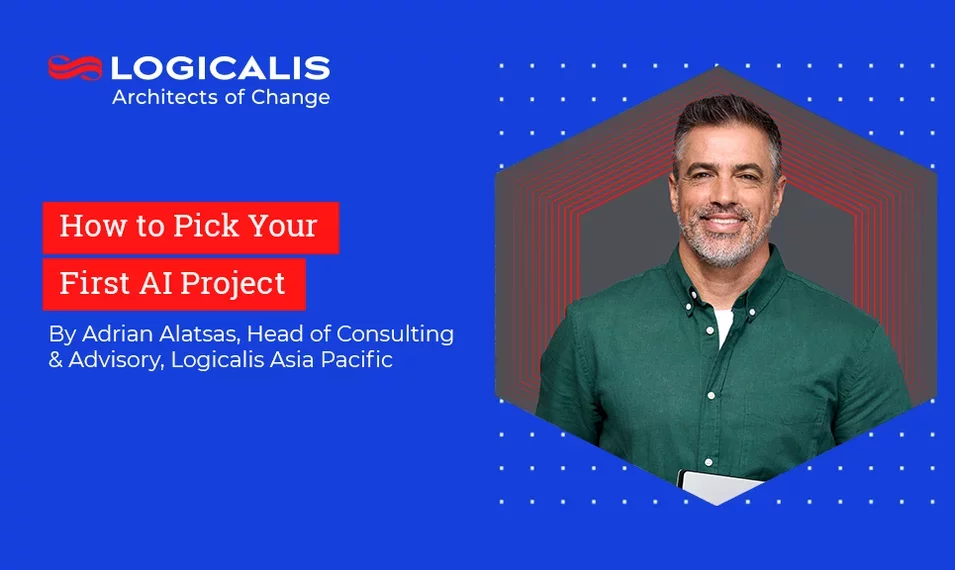
Australia , Apr 3, 2025
How to Pick Your First AI Project
By Adrian Alatsas, Head of Consulting & Advisory, Logicalis Asia Pacific
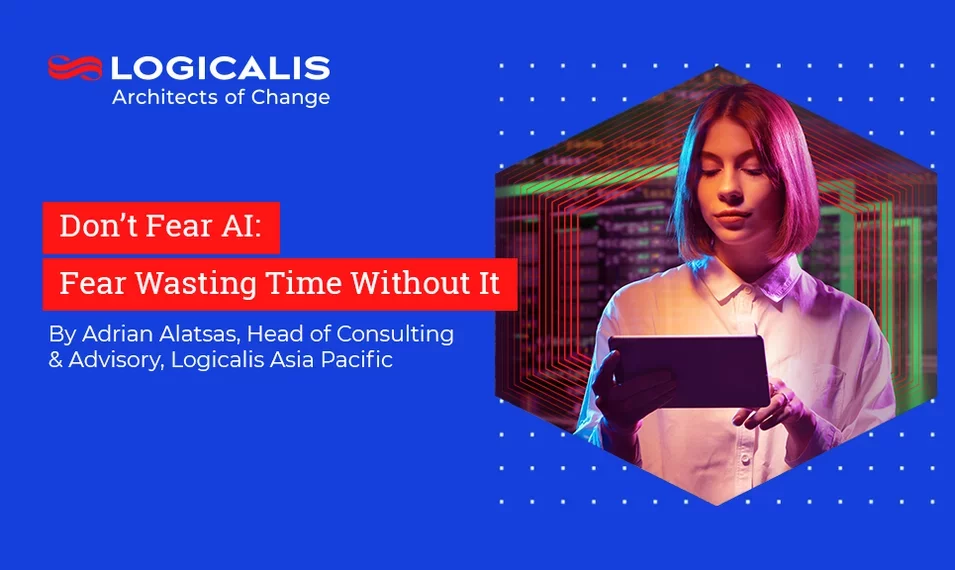
Australia , Apr 3, 2025
Don’t Fear AI: Fear Wasting Time Without It
By Adrian Alatsas, Head of Consulting & Advisory, Logicalis Asia Pacific
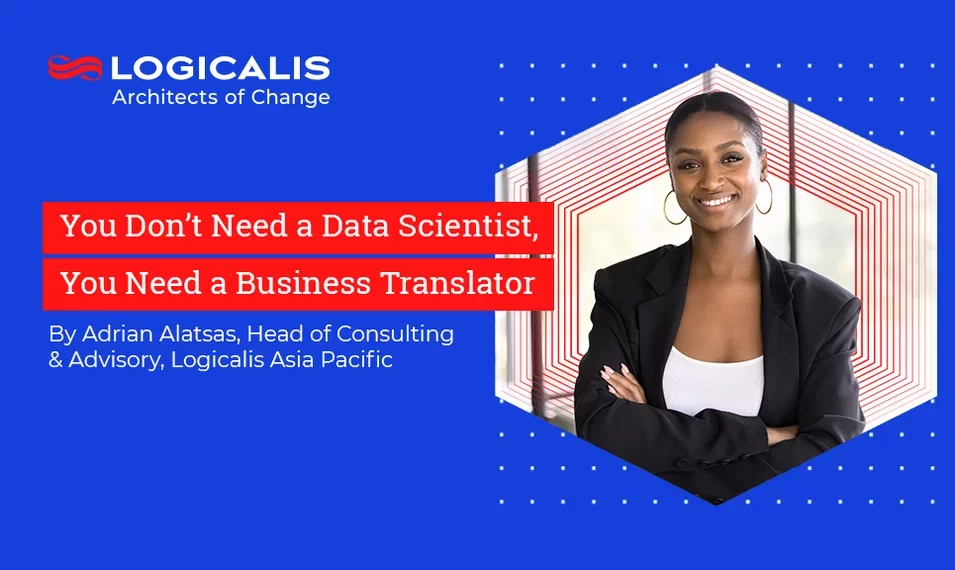
Australia , Apr 3, 2025
You Don’t Need a Data Scientist, You Need a Business Translator
By Adrian Alatsas, Head of Consulting & Advisory, Logicalis Asia Pacific

Australia , Apr 3, 2025
The Real ROI of AI Isn’t Just Cost Saving
By Adrian Alatsas, Head of Consulting & Advisory, Logicalis Asia Pacific
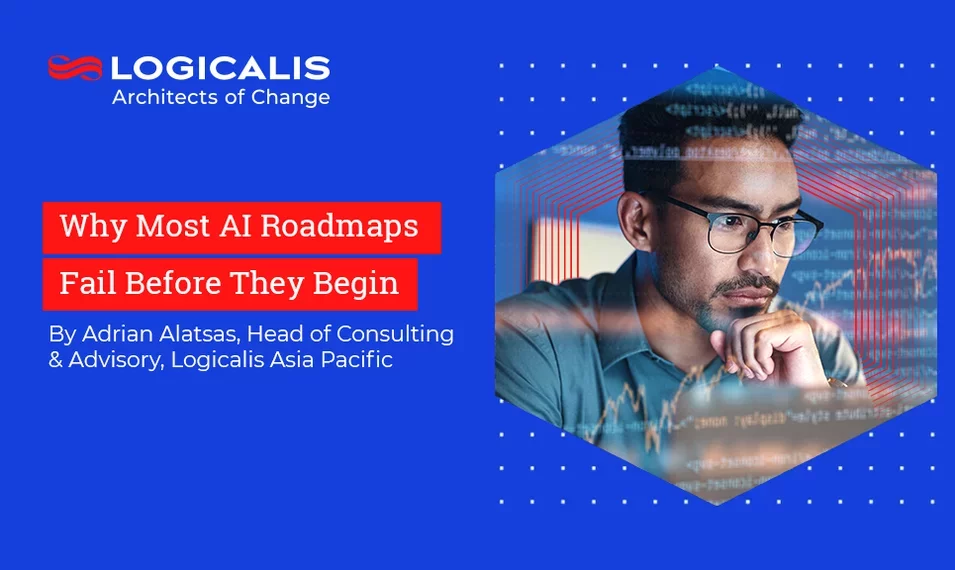
Australia , Apr 3, 2025
Why Most AI Roadmaps Fail Before They Begin
By Adrian Alatsas, Head of Consulting & Advisory, Logicalis Asia Pacific
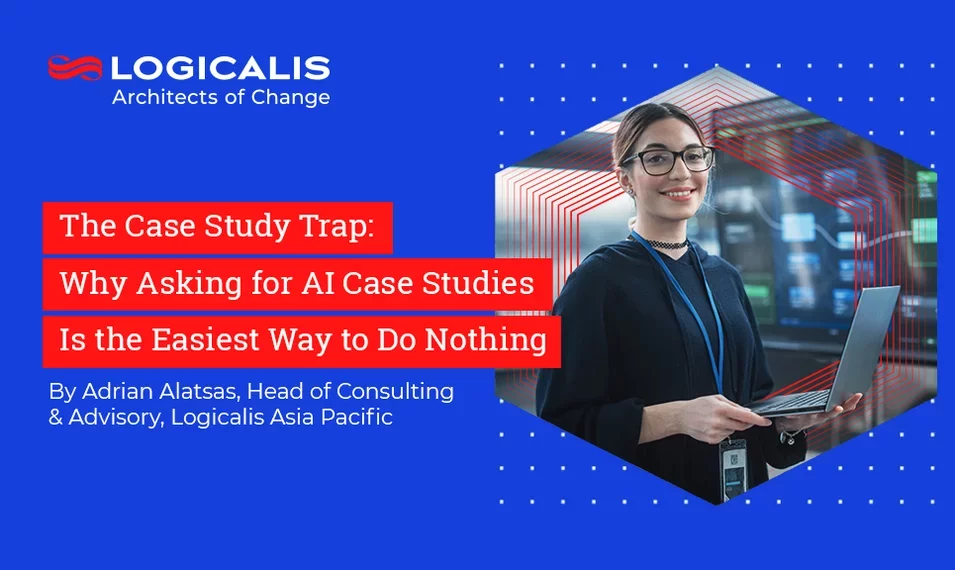
Australia , Apr 3, 2025
The Case Study Trap: Why Asking for AI Case Studies Is the Easiest Way to Do Nothing
By Adrian Alatsas, Head of Consulting & Advisory, Logicalis Asia Pacific
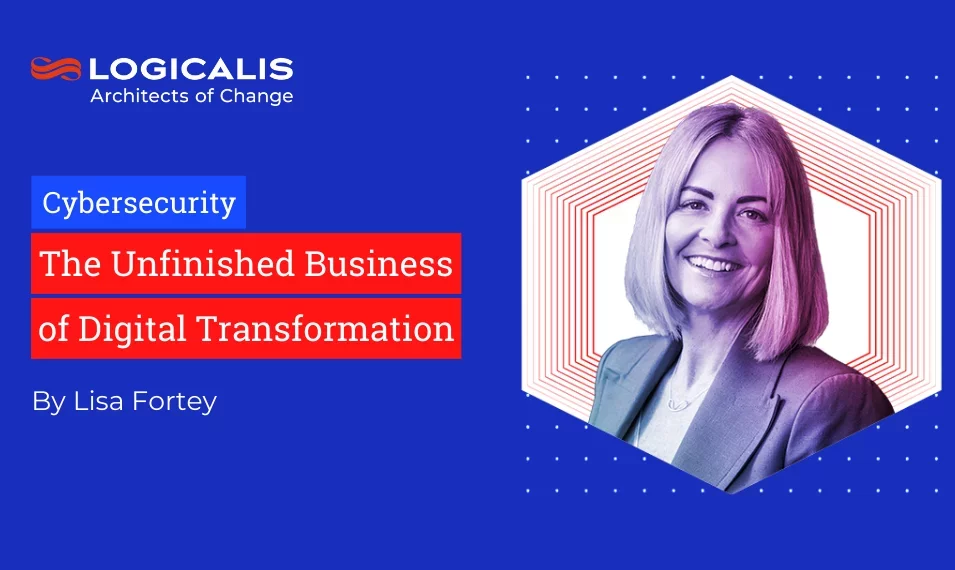
Australia , Apr 1, 2025
Cybersecurity – The Unfinished Business of Digital Transformation
If you’ve followed this blog series from the start—thank you for staying with us as we unpack the 2025 Australian federal budget’s impact on technology investment. In Blog 1, we explored how AI, cloud, and cybersecurity are driving digital transformation, aligning with key investment trends from the Logicalis APAC CIO Report and ADAPT’s Top 10 CIO Priorities for 2025. In Blog 2, we examined AI adoption beyond the hype, showcasing real-world use cases across industries and tackling common implementation challenges.
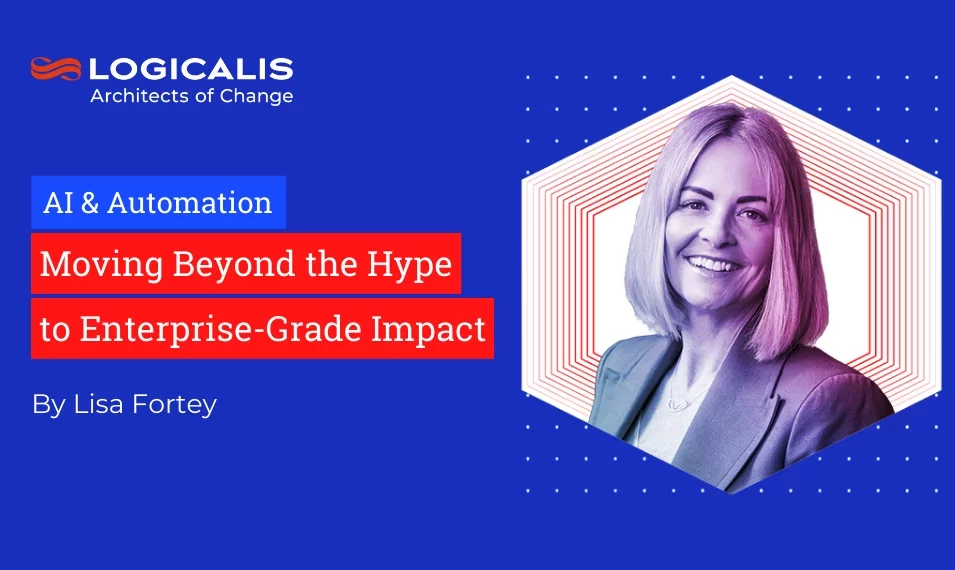
Australia , Apr 1, 2025
AI & Automation – Moving Beyond the Hype to Enterprise-Grade Impact
investment in key technology areas—AI, cybersecurity, cloud infrastructure, and workforce development. These initiatives align closely with Logicalis’ APAC CIO Report and ADAPT’s Top 10 CIO Priorities for 2025, where CIOs are prioritizing data strategy, security, and AI adoption. While AI & automation have received substantial funding, many organisations struggle to move beyond experimentation and deploy AI at an enterprise scale. So, how can CIOs turn budget-driven AI initiatives into real business value?
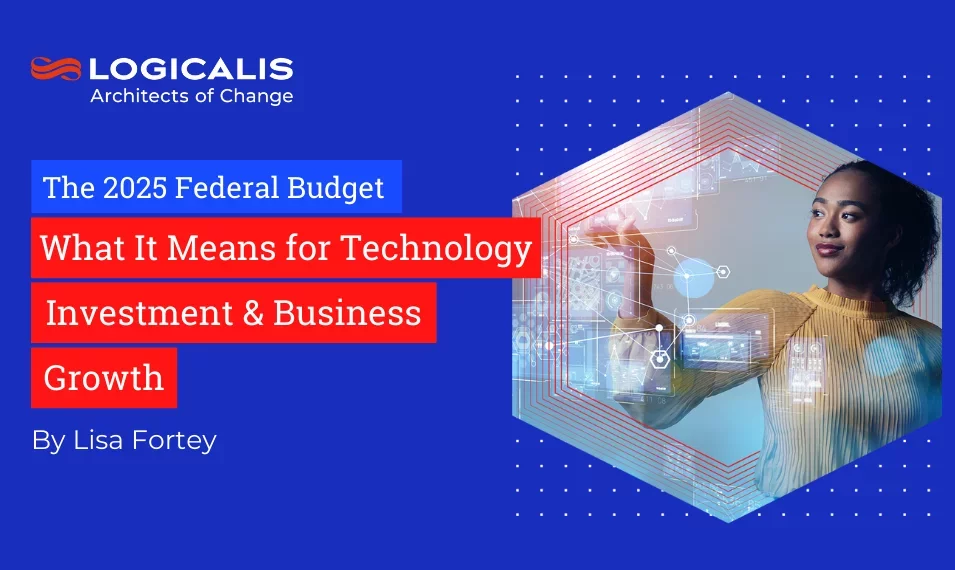
Australia , Mar 31, 2025
The 2025 Federal Budget – What It Means for Technology Investment & Business Growth
Drawing insights from Logicalis’ APAC CIO Report and ADAPT’s Top 10 CIO Priorities for 2025, this blog will explore what the budget means for IT leaders and how Logicalis can help these CIOs navigate the road ahead.
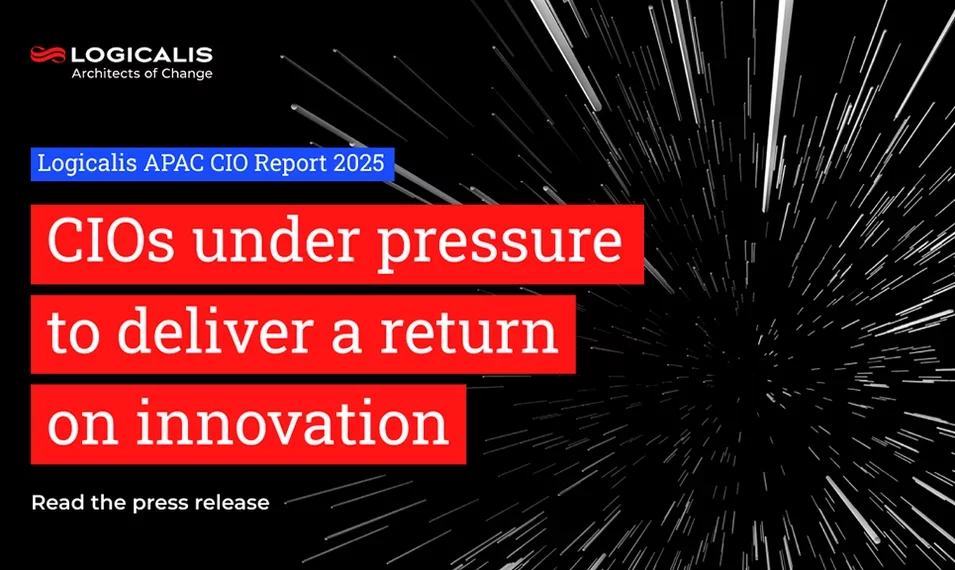
Australia , Mar 17, 2025
Eleventh annual Logicalis CIO Report 2025 finds 96 per cent of APAC CIOs are prioritising technology to drive new revenue streams
Logicalis, the leading global technology service provider, has released its annual CIO Report, which reveals that 95% of organisations are actively investing in technology to create new revenue streams within the next 12 months.
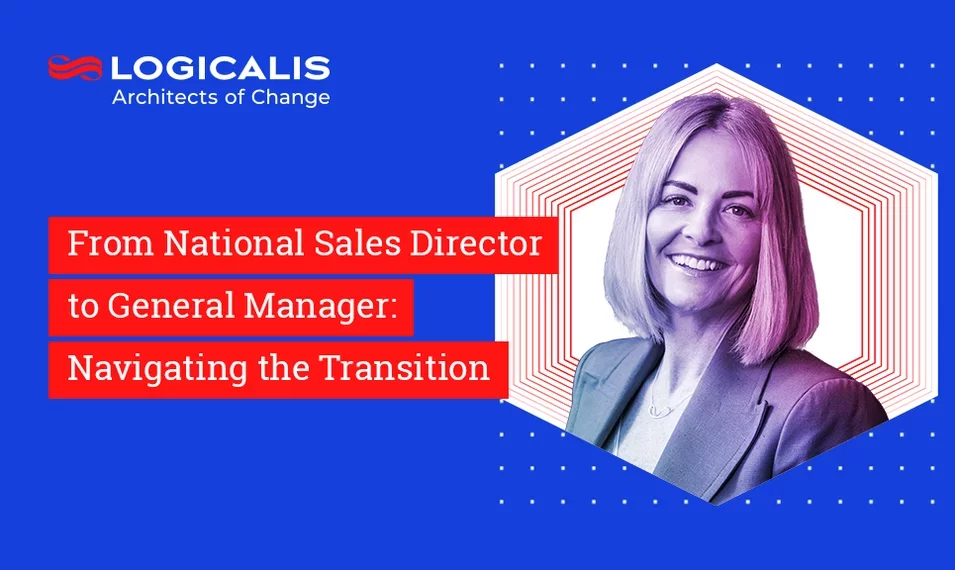
Australia , Feb 21, 2025
From National Sales Director to General Manager: Navigating the Transition
Stepping into the Acting General Manager (GM) role, from a National Sales Director position has been both an exciting and challenging transition. While sales leadership equips you with strategic thinking, relationship management, and revenue growth skills, the GM role demands a broader business perspective. Here’s what I’ve learned on my journey so far.
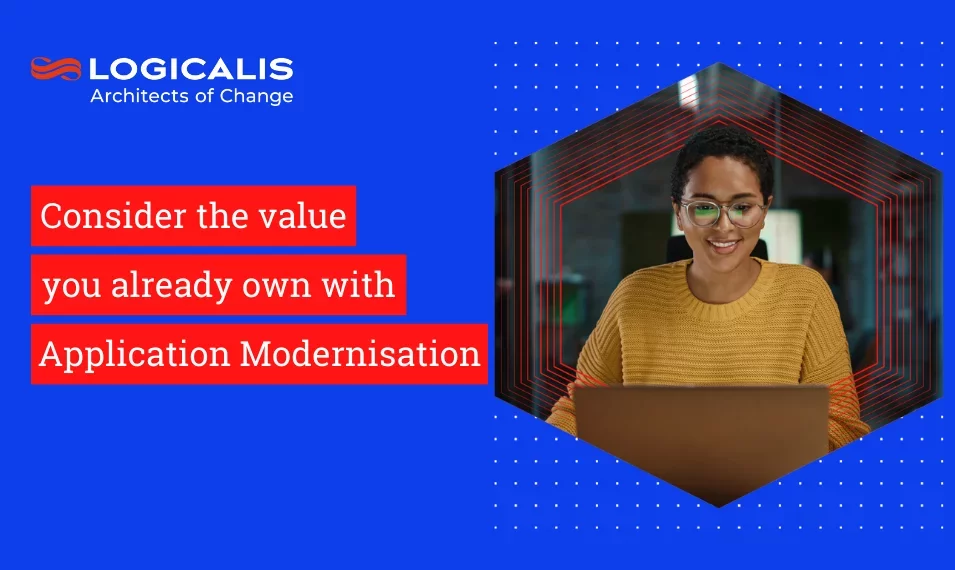
Australia , Feb 12, 2025
Consider the value you already own with Application Modernisation
Application modernisation, often overlooked in digital transformation, can be a cost-effective way to leverage the benefits of cloud technology. This involves updating legacy applications to improve performance, security, and efficiency, allowing for greater scalability, flexibility, and integration.
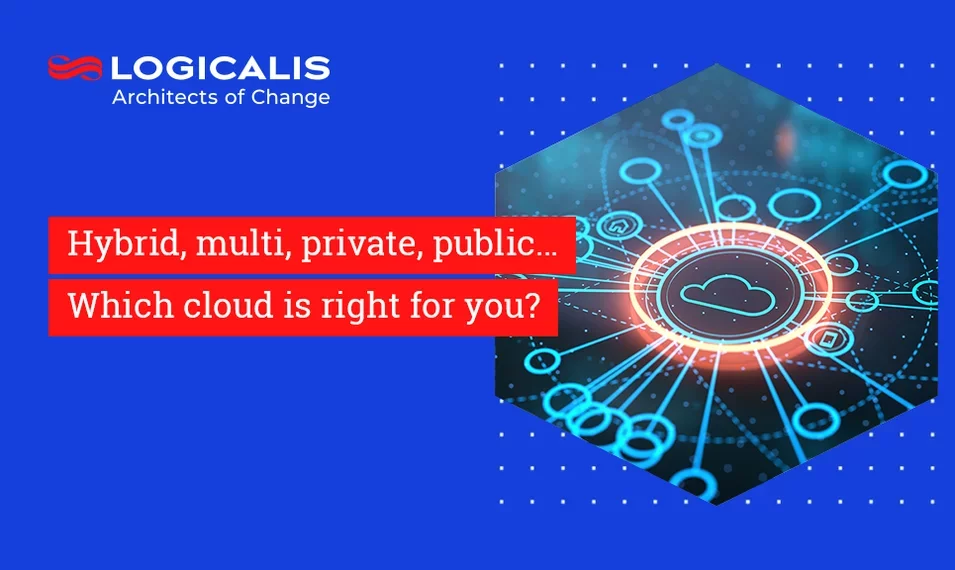
Australia , Feb 12, 2025
Hybrid, multi, private, public… Which cloud is right for you?
Australian organisations are increasingly adopting cloud strategies as part of their technology infrastructure. The Australian Bureau of Statistics surveyed Australian businesses in 2022 and found that 85% were using ICT1, with 59% of these using cloud technology. Gartner predicts that cloud computing will become a business necessity by 2028.
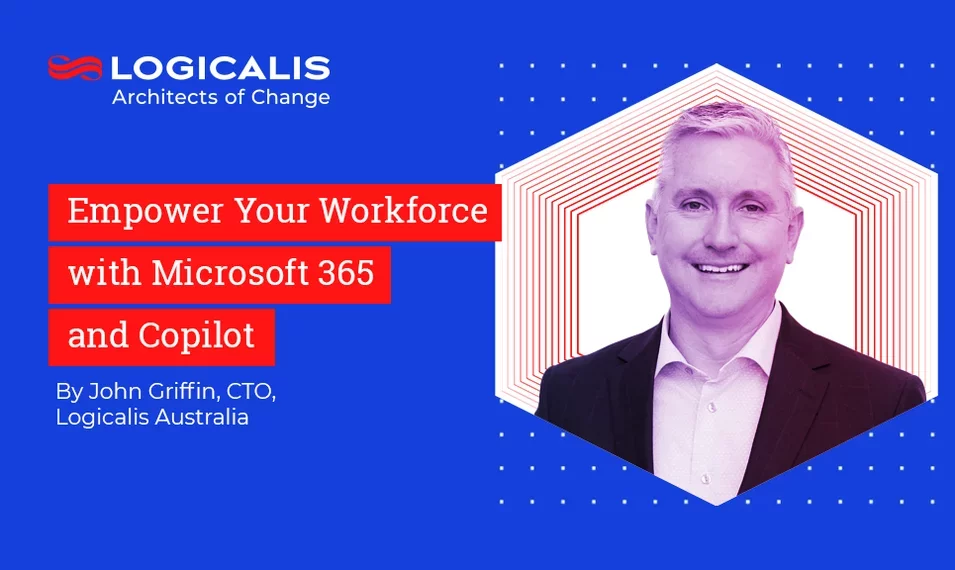
Australia , Jan 15, 2025
Empower Your Workforce with Microsoft 365 and Copilot
A feeling of wonder and marvel can happen with new technologies when you suddenly realise that all the hype and promises are real. Around the world, we’re seeing this right now with generative AI, which has whipped up a lot of hype in such a short time. As organisations come to terms with generative AI and how it can revolutionise their business, they can be left thinking about when and how to modernise their workforce best.
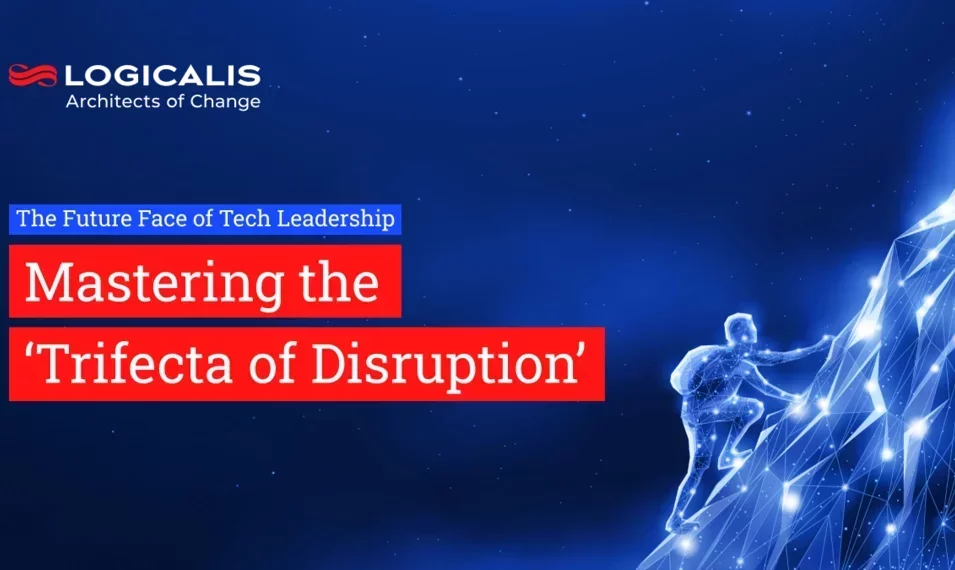
Australia , Jan 8, 2025
Future Face of Tech Leadership: Mastering the ‘Trifecta of Disruption’
Bailkoski highlights three standout themes from Logicalis Group’s latest CIO Report—all anchored in disruption. As CIOs and tech leaders navigate these rapid shifts, the annual CIO Report sheds light on the evolving priorities and challenges facing IT leadership today. Addressing a room of industry experts, Bailkoski reflected on how the role of IT leadership has transformed over the past decade. A decade of evolution: From shadow IT to digital transformation. Bailkoski recalled 2014’s key theme: ‘Shadow IT.’
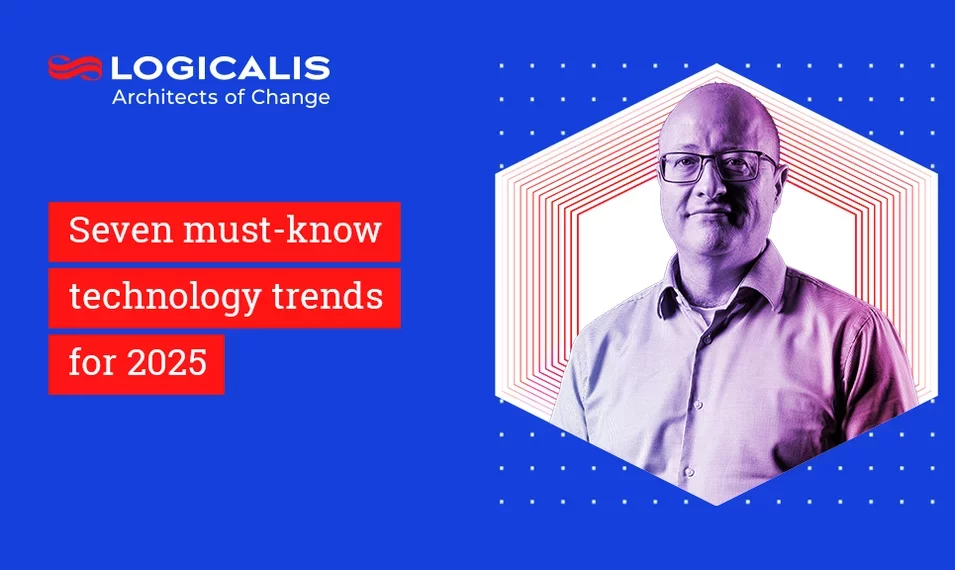
Australia , Dec 9, 2024
Seven must-know technology trends for 2025
Technological innovation is reshaping business competition rules. Yesterday’s successful strategies may not be enough for tomorrow’s challenges and businesses must adapt to the next wave of advancements, from quantum computing to industry-specific artificial intelligence (AI) solutions. Logicalis Australia has identified seven key trends for organisations to stay ahead in 2025.
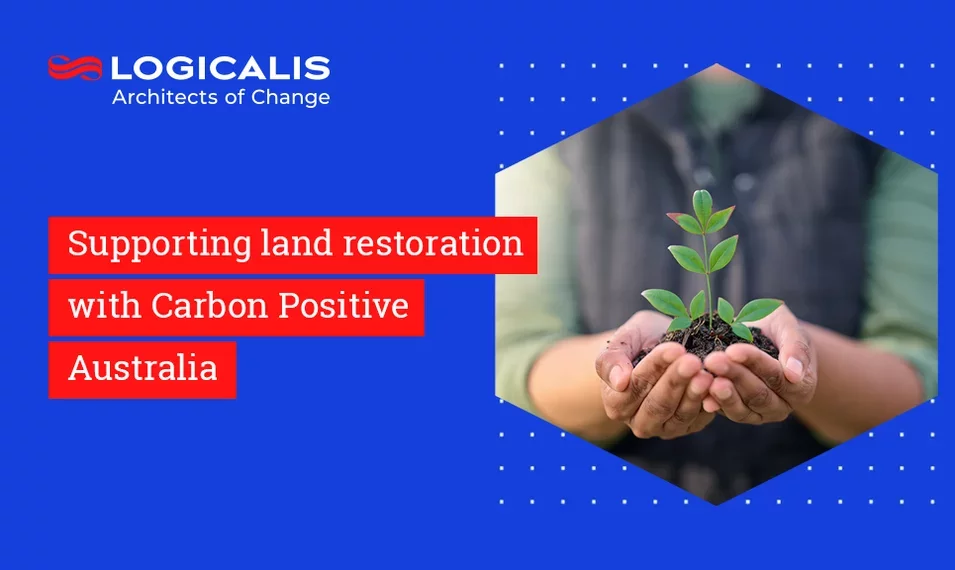
Australia , Nov 26, 2024
Supporting land restoration with Carbon Positive Australia
In 2023, Logicalis made a commitment to playing a proactive role in environmental sustainability by partnering with Carbon Positive Australia to restore land and biodiversity across the country. As part of our sustainability pledge, for every badge scanned at our events and for every attendee at our exclusive events, we fund the planting of native trees throughout Australia. This initiative has resulted in the planting of over 2,700 native trees, helping to offset carbon while supporting habitat restoration and biodiversity.
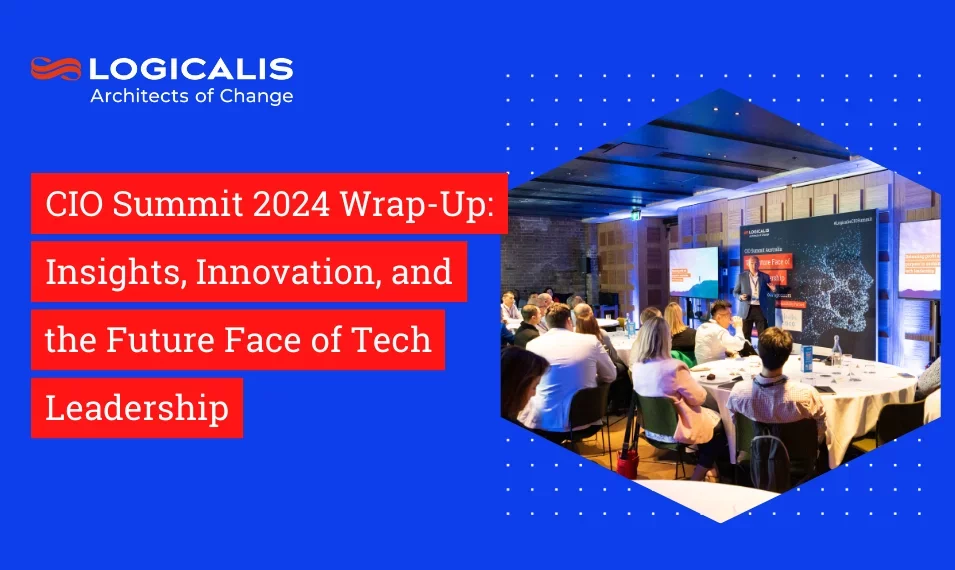
Australia , Nov 21, 2024
CIO Summit 2024 Wrap-Up: Insights, Innovation, and the Future Face of Tech Leadership
Last month wrapped up the end of the Logicalis global CIO Summit roadshow, culminating in Australia where events were held in both Sydney and Melbourne.
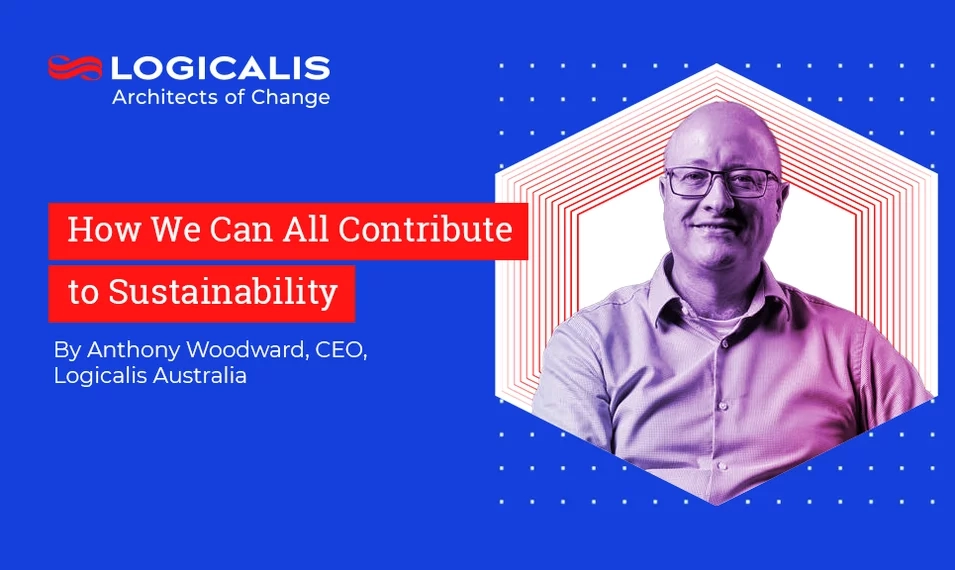
Australia , Nov 13, 2024
Creating Change: How We Can All Contribute to Sustainability
Last month, our global CEO, Bob Bailkoski, visited Sydney and Melbourne for the final two events on the global CIO Summit Roadshow. In his keynote, Bob reflected on a decade of the Logicalis CIO Report, tracing the journey from 2014's "shadow IT" to today's complex landscape of technological growth, cybersecurity, and increasing regulatory and sustainability demands.
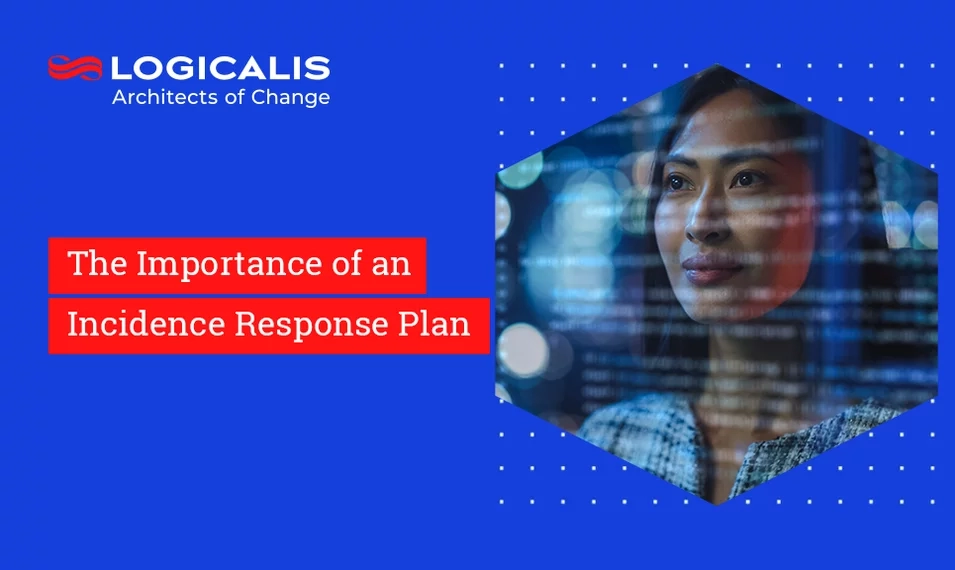
Australia , Nov 11, 2024
The Importance of an Incidence Response Plan
In today’s interconnected world, service outages can have serious repercussions for any organisation, affecting operations, customer trust, and even compliance with regulations. Without a clear plan, teams may make rushed decisions under pressure, leading to greater disruptions or prolonged recovery times.
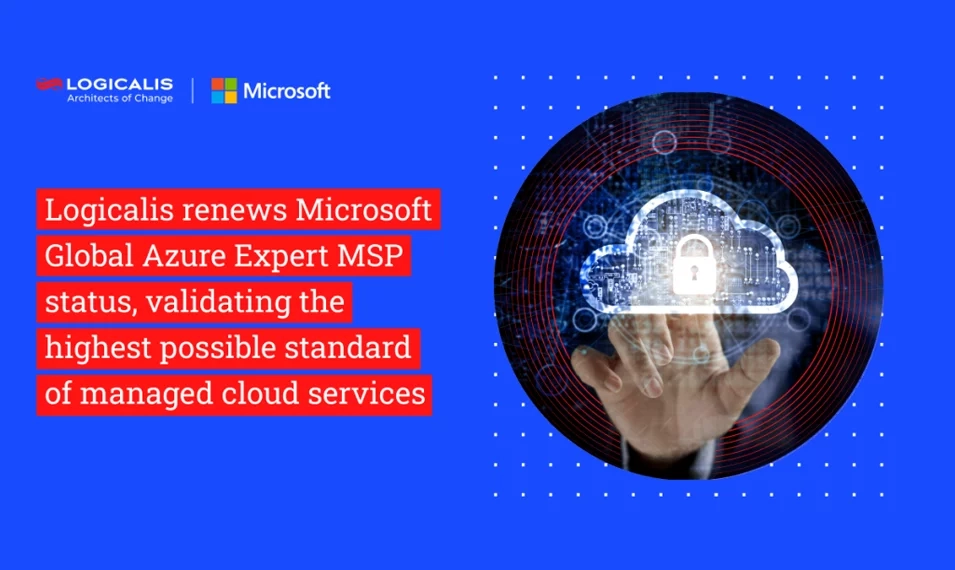
APAC , Nov 6, 2024
Logicalis renews Microsoft Global Azure Expert MSP status, validating the highest possible standard of managed cloud services.
Logicalis are proud to announce they have renewed the prestigious Global Azure Expert Managed Service Provider (AEMSP) certification. This accolade not only showcases Logicalis’ in-depth knowledge and proficiency in deploying, managing, and optimising Azure-based solutions but also emphasises their ability to deliver transformative outcomes for clients.
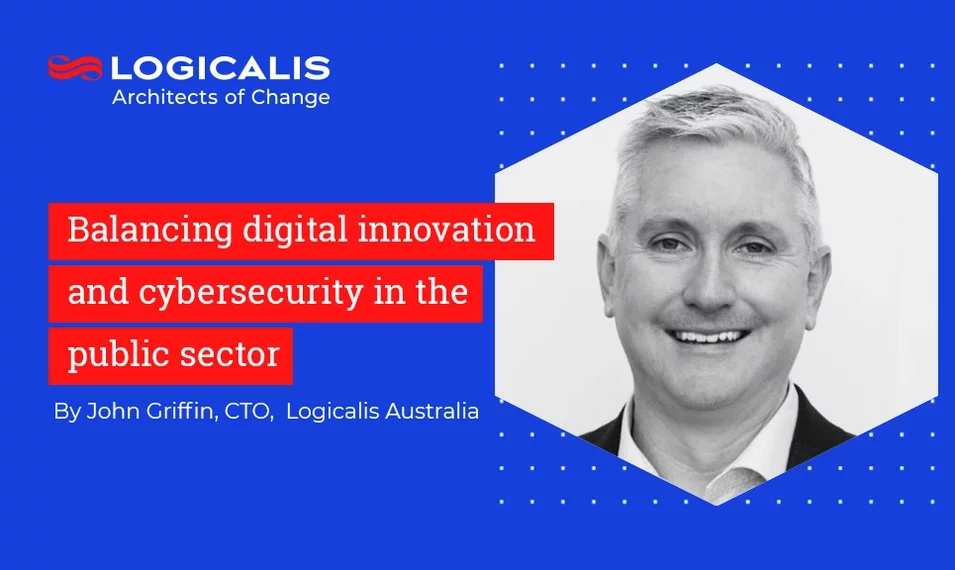
Australia , Nov 5, 2024
Balancing digital innovation and cybersecurity in the public sector
Balancing risk with digital innovation is a critical priority for government departments, agencies, and organisations as they navigate the complexities of digital transformation.
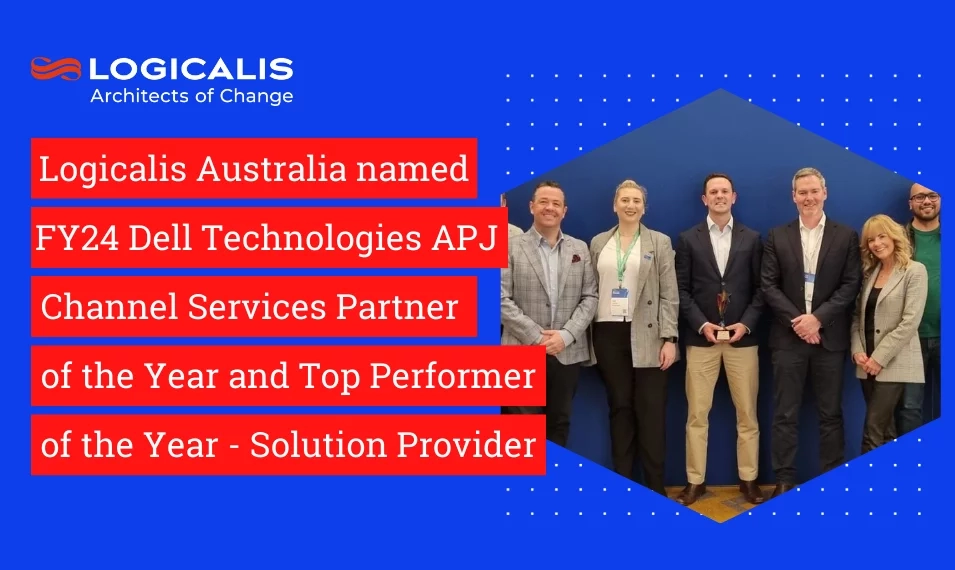
Australia , Oct 31, 2024
Logicalis Australia named FY24 Dell Technologies APJ Channel Services Partner of the Year and Top Performer of the Year – Solution Provider
Logicalis Australia is thrilled to have been named FY24 Dell Technologies Asia Pacific and Japan (APJ) Channel Services Partner of the Year and also recognised as the Top Performer of the Year – Solution Provider.
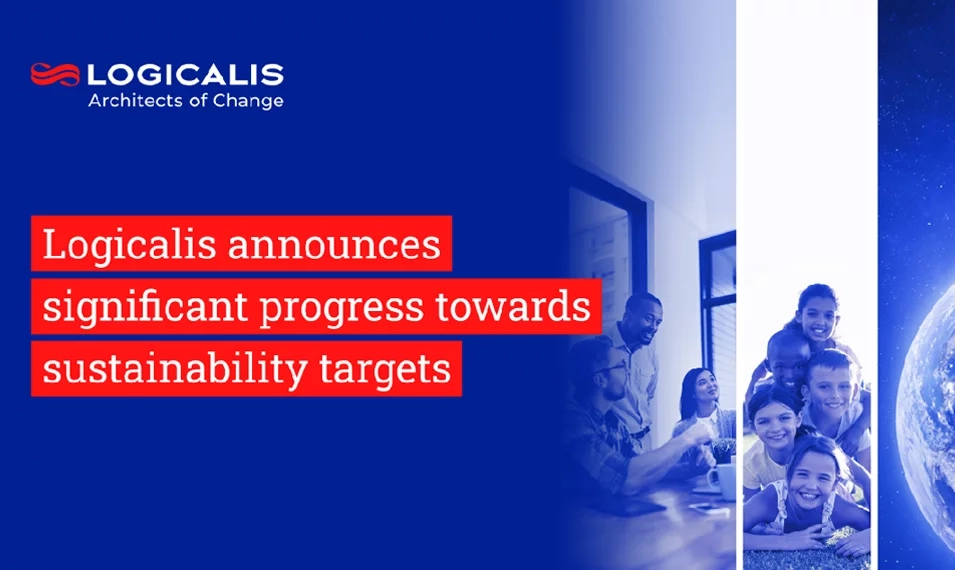
APAC , Oct 29, 2024
Logicalis recognised as Global Sustainability Partner of the Year, for the second consecutive year at Cisco Partner Summit 2024
Logicalis receives the global accolade, in recognition of its leadership and performance in sustainable practices for the second year running
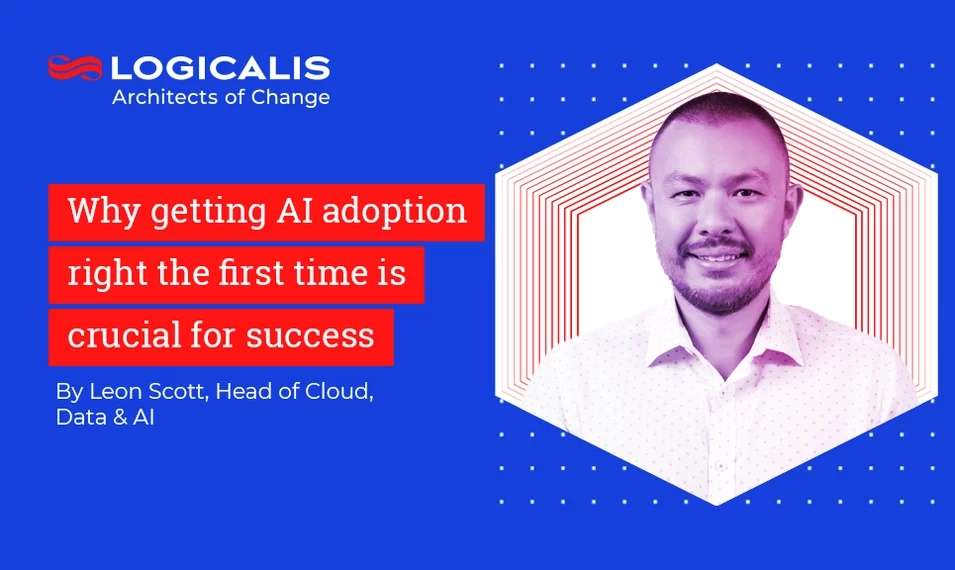
Australia , Oct 25, 2024
Why getting AI adoption right the first time is crucial for success
The potential of AI is vast, offering opportunities to enhance efficiency, drive innovation, and reshape entire sectors.
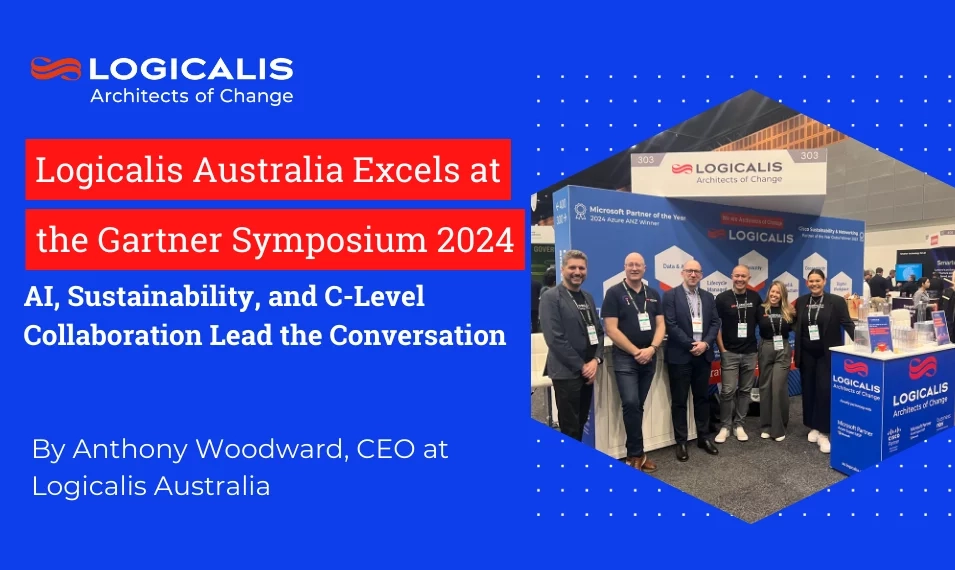
Australia , Oct 21, 2024
Logicalis Australia Excels at Gartner Symposium 2024: AI, Sustainability, and C-Level Collaboration Lead the Conversation
I recently attended Gartner Symposium 2024 on the Gold Coast, with Logicalis Australia there in force over the three days to build our brand and have plenty of engaging conversations with customers and prospects.
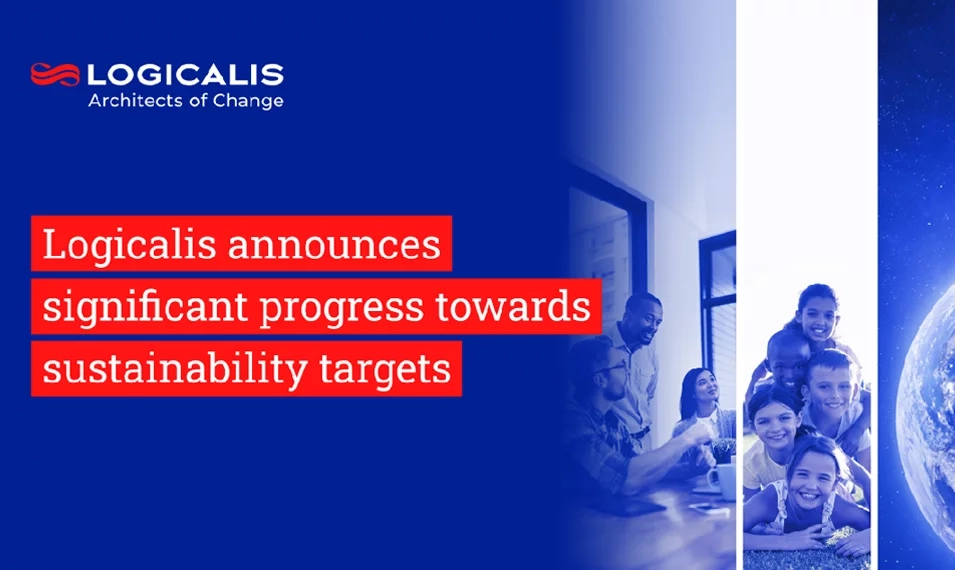
Indonesia , Sep 17, 2024
Logicalis announces significant progress towards sustainability targets
Logicalis, a global technology service provider, has announced significant progress towards its sustainability targets in the Logicalis Responsible Business Report 2024. The business reported a 27% reduction in scope 1 emissions since FY22, while market-based scope 2 emissions were reduced by 17%.
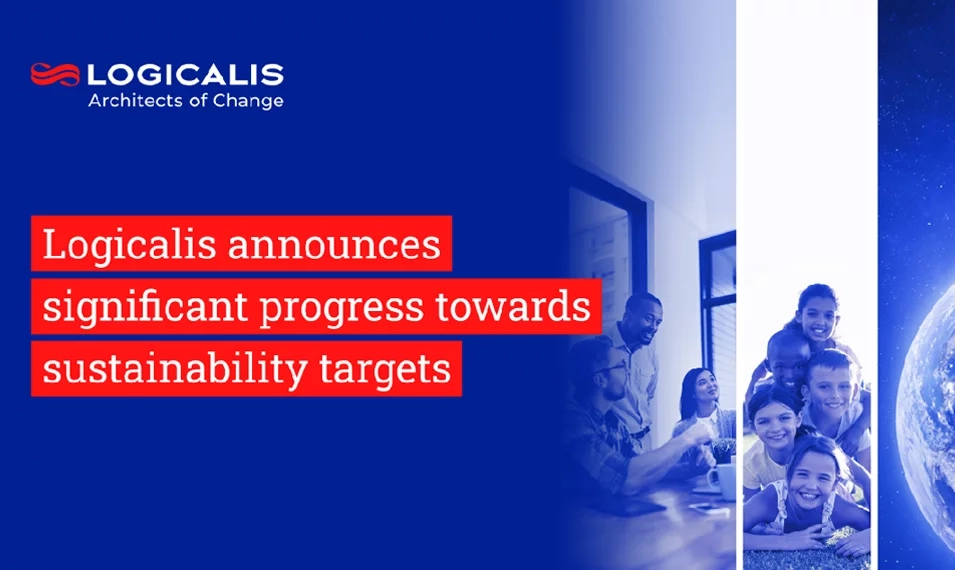
APAC , Sep 13, 2024
Logicalis announces significant progress towards sustainability targets
Logicalis, a global technology service provider, has announced significant progress towards its sustainability targets in the Logicalis Responsible Business Report 2024. The business reported a 27% reduction in scope 1 emissions since FY22, while market-based scope 2 emissions were reduced by 17%.
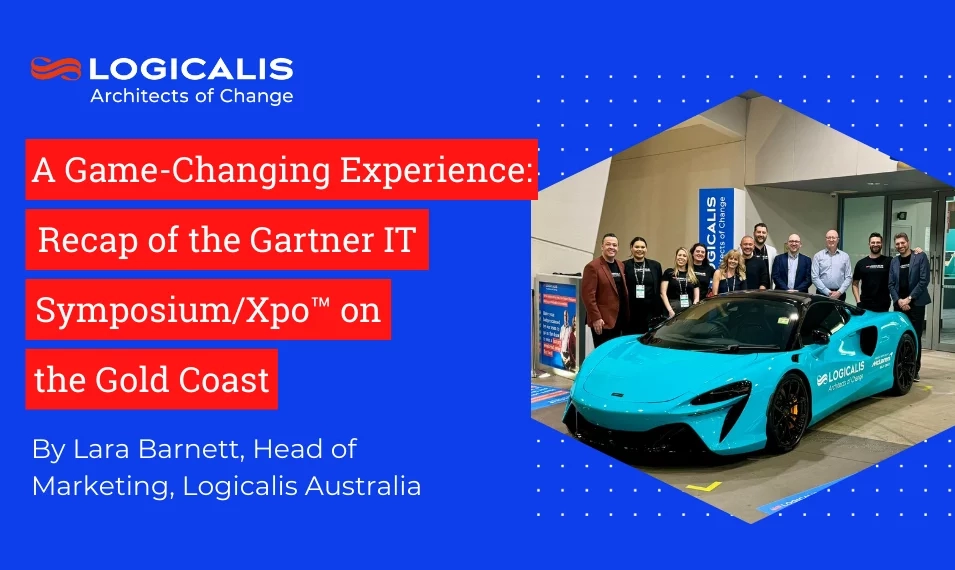
Australia , Sep 13, 2024
A Game-Changing Experience: Recap of the Gartner IT Symposium/Xpo™ on the Gold Coast
From September 9th to 11th, the Gartner IT Symposium on the Gold Coast brought together over 2,000 IT professionals, including a large contingent from Logicalis Australia.
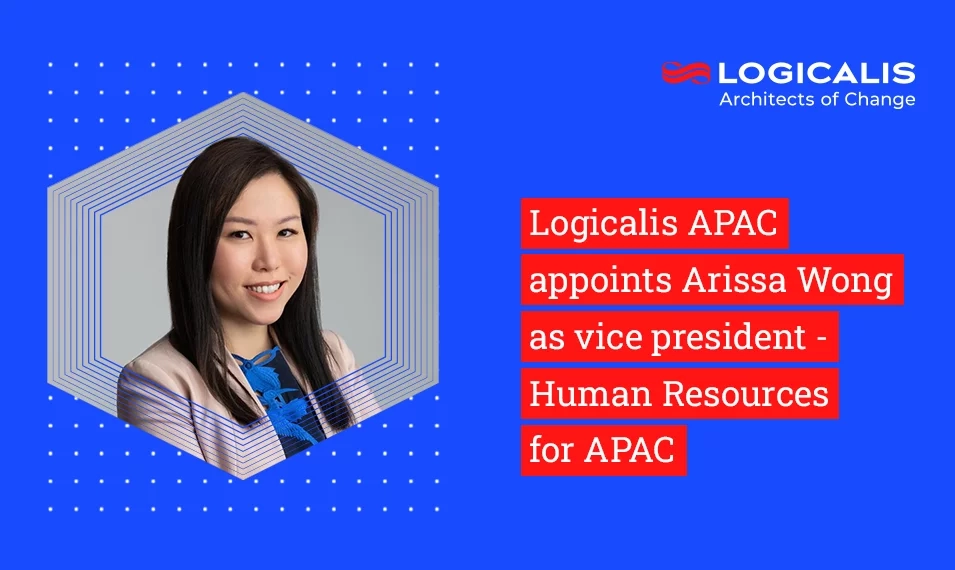
APAC , Sep 9, 2024
Logicalis APAC appoints Arissa Wong as vice president – Human Resources for APAC
Logicalis, a leading provider of global IT solutions and managed services, has appointed Arissa Wong as Vice President –Human Resources (HR) for the Asia Pacific (APAC) region, effective immediately. This newly formed role follows the departure of Scott Brown, the previous head of Employee Experience for Logicalis Australia.
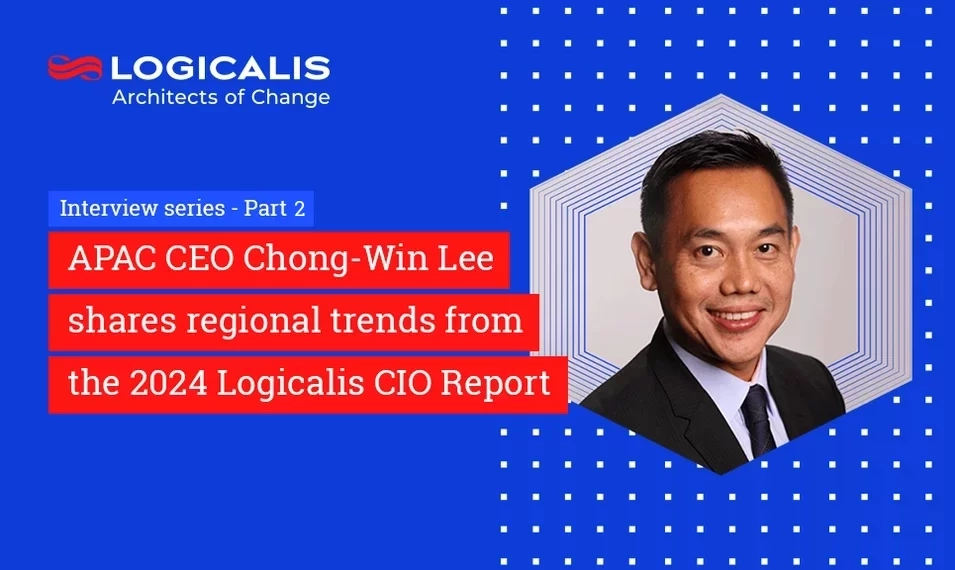
Australia , Sep 8, 2024
Interview series - Part 2: APAC CEO Chong-Win Lee shares regional trends from the 2024 Logicalis CIO Report
Chong-Win Lee (Win) recently became CEO of Logicalis Asia Pacific, after the company combined its Asian and Australian operations in May 2024.
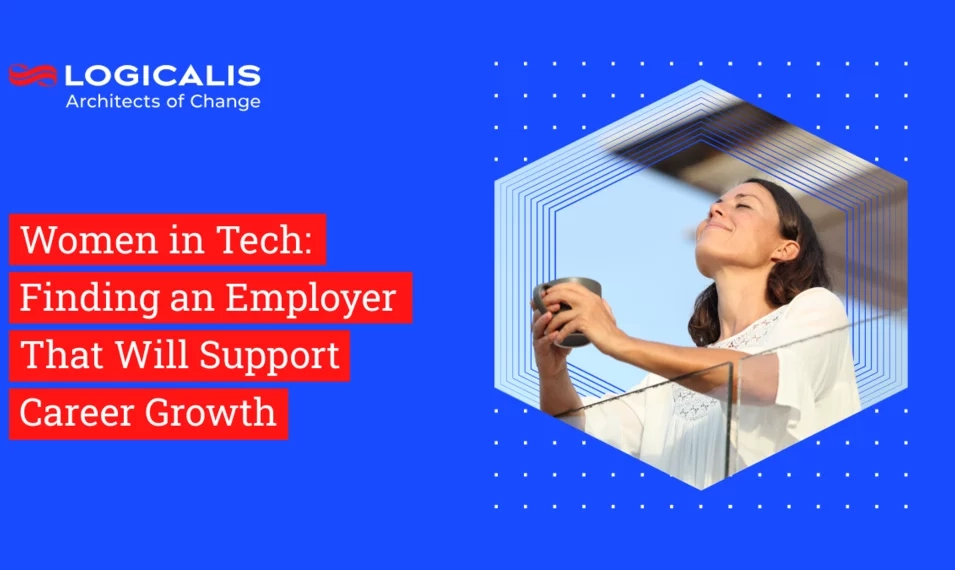
Australia , Aug 5, 2024
Women in tech: Finding an employer that will support career growth
In her latest article, Dina Knight, Chief People Office at Logicalis shares her insights on what women should look out for when considering organisations that will support their career growth. She draws on her experience at Logicalis to outline her top tips, stretching beyond the broad focus on training and skills development.
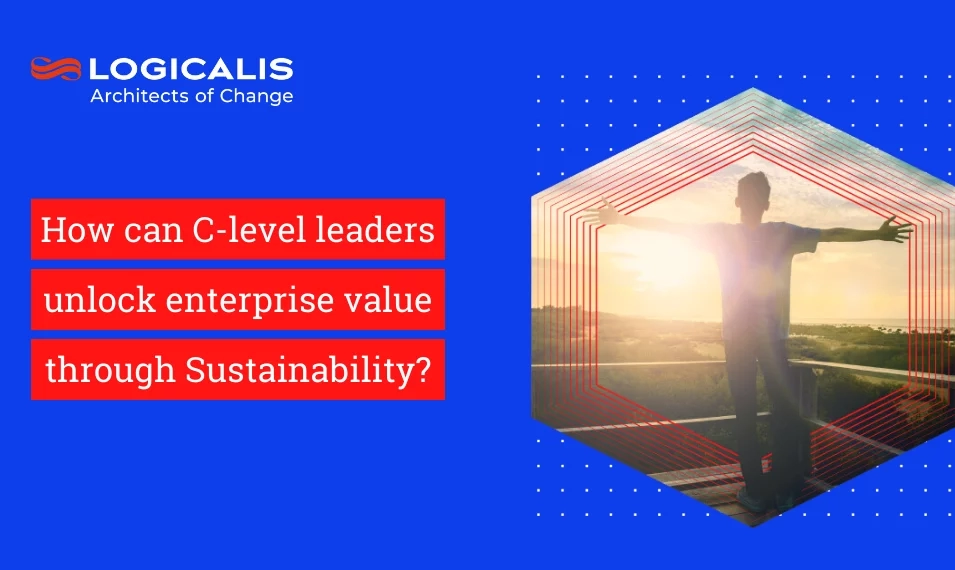
Australia , Aug 5, 2024
How can C-level leaders unlock enterprise value through Sustainability?
Sustainability has become a crucial factor in driving enterprise value, but how can C-level executives leverage sustainability to enhance their organisation’s performance, compliance and competitive edge?
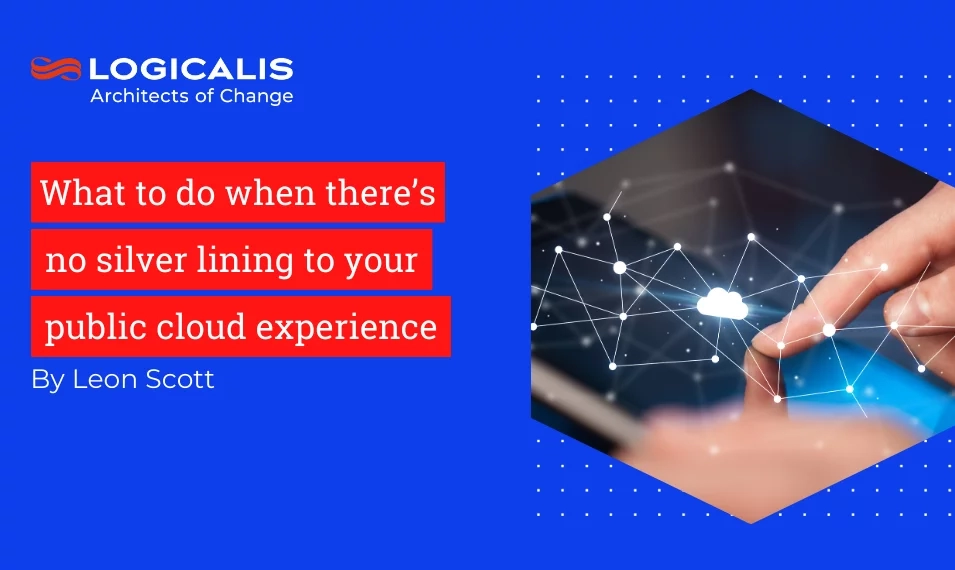
Australia , Jul 30, 2024
What to do when there’s no silver lining to your public cloud experience
Like many Australian companies recently, your IT team may have shifted from on-premises infrastructure or hosted data centres across to public cloud to access the many benefits of hyperscale cloud computing. While this shift has helped to bring processes online and empower a hybrid workforce with computing power at scale, in some cases it has led to unexpected bill shock each month.
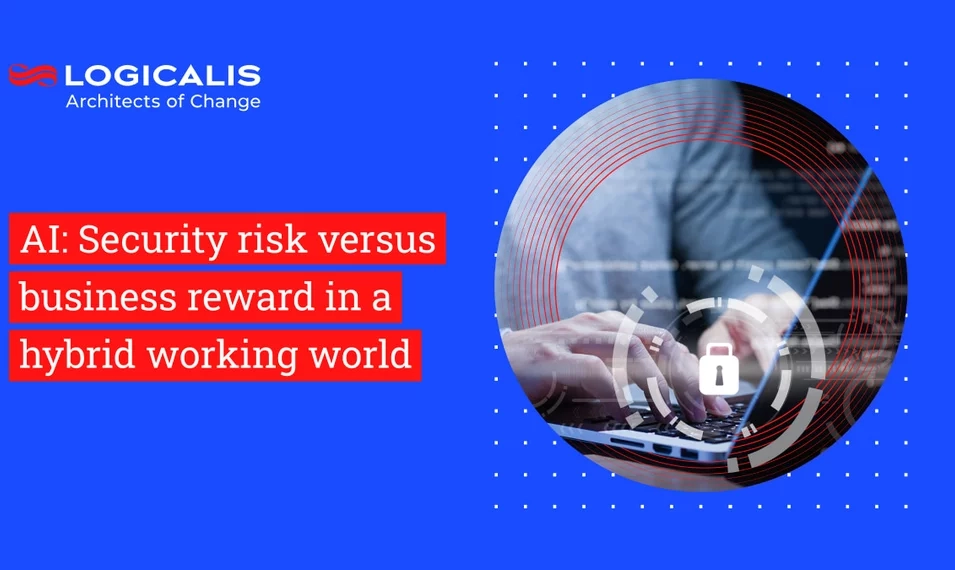
Australia , Jul 25, 2024
AI: Security risk versus business reward in a hybrid working world
Protect your organisation from cyber threats with AI powered security solutions. Enable flexible working while ensuring data privacy and protection. Read Bob Bailkoski's article originally published in Forbes.
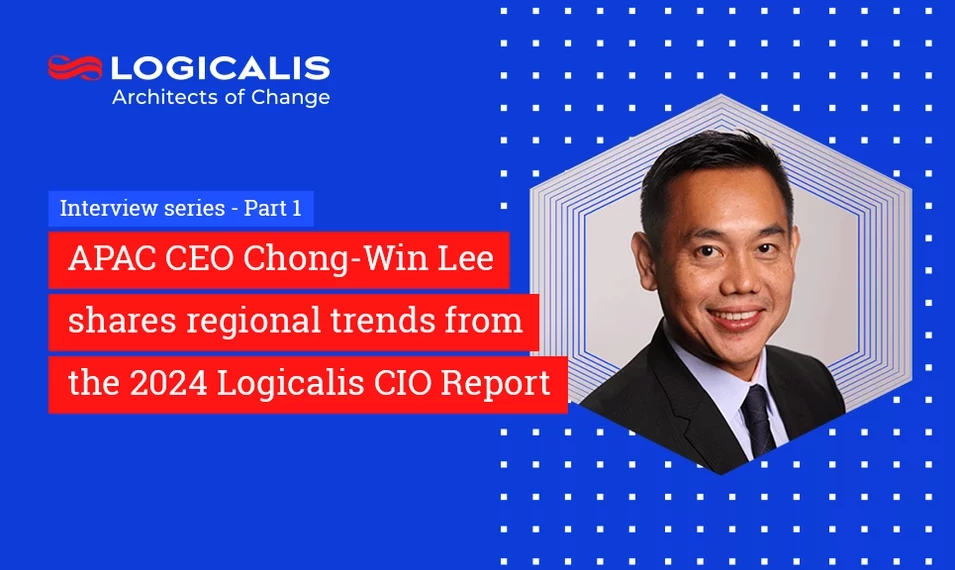
Australia , Jul 16, 2024
Interview series - Part 1: APAC CEO Chong-Win Lee shares regional trends from the 2024 Logicalis CIO Report
Chong-Win Lee (Win) recently became CEO of Logicalis Asia Pacific, after the company combined its Asian and Australian operations in May 2024.
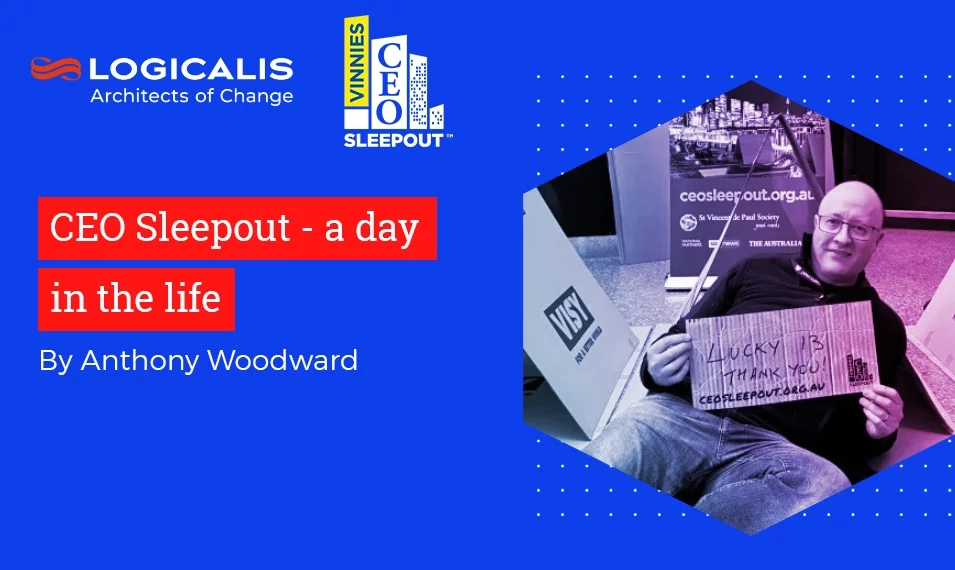
Australia , Jun 26, 2024
CEO Sleepout - A day in the life
IoT technologies, AI, and advanced data analytics are shaping up as business-critical solutions that can give Australian companies a significant competitive edge.
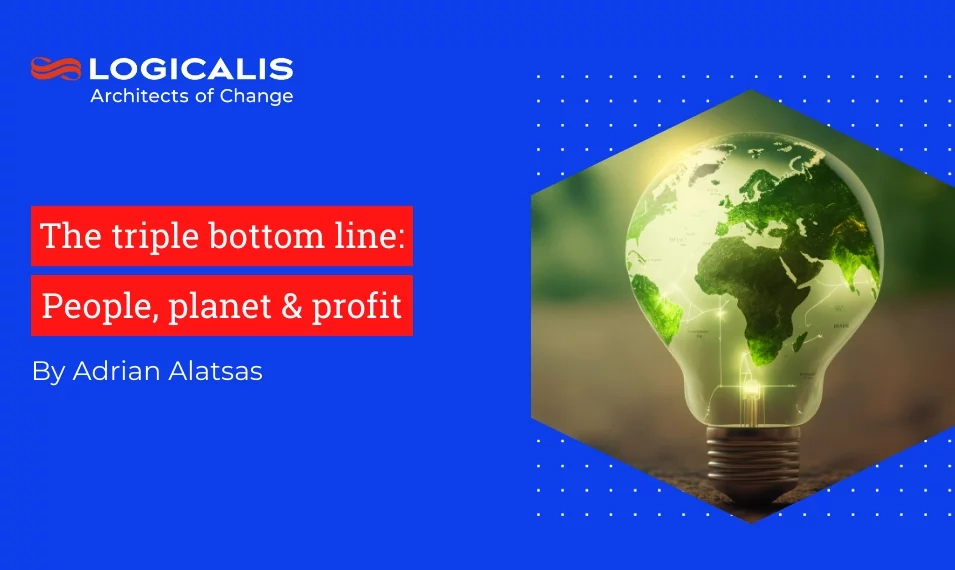
Australia , May 29, 2024
The triple bottom line: People, planet & profit
In today's fast changing business world, sustainability isn’t just an option – it’s a must for all industries, including IT. Leading the charge in sustainability not only benefits the planet but it also affects people and profits, aligning with the concept of the triple bottom line.
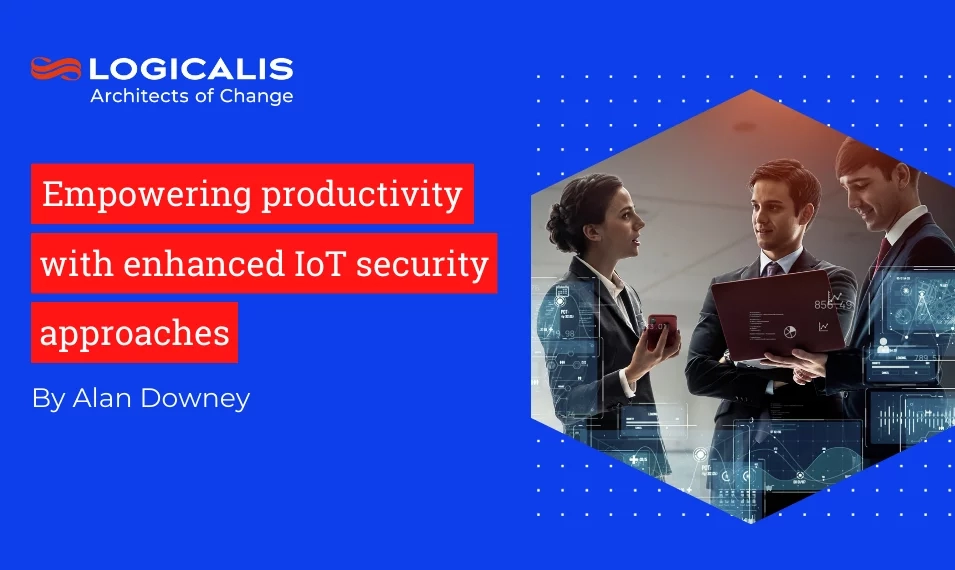
Australia , May 29, 2024
Empowering productivity with enhanced IoT security approaches
IoT technologies, AI, and advanced data analytics are shaping up as business-critical solutions that can give Australian companies a significant competitive edge.
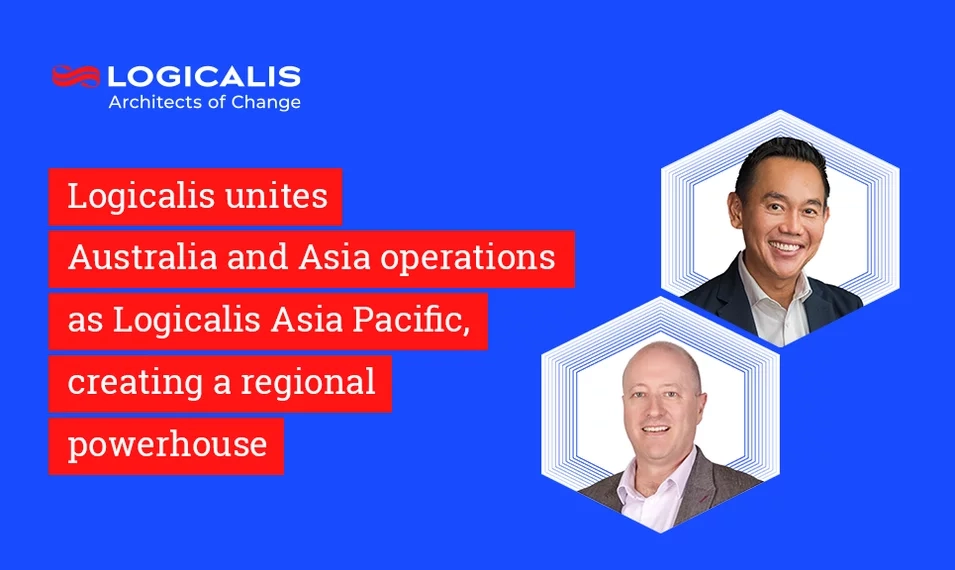
Australia , May 8, 2024
Logicalis unites Australia and Asia operations as Logicalis Asia Pacific, creating a regional powerhouse
Logicalis, a leading global technology services provider, has announced the creation of a new Asia Pacific entity, combining its Logicalis Australia and Logicalis Asia operations. This strategic realignment will be effective from June 1, 2024, and positions Logicalis to amplify its market presence through increased synergy within the APAC region.
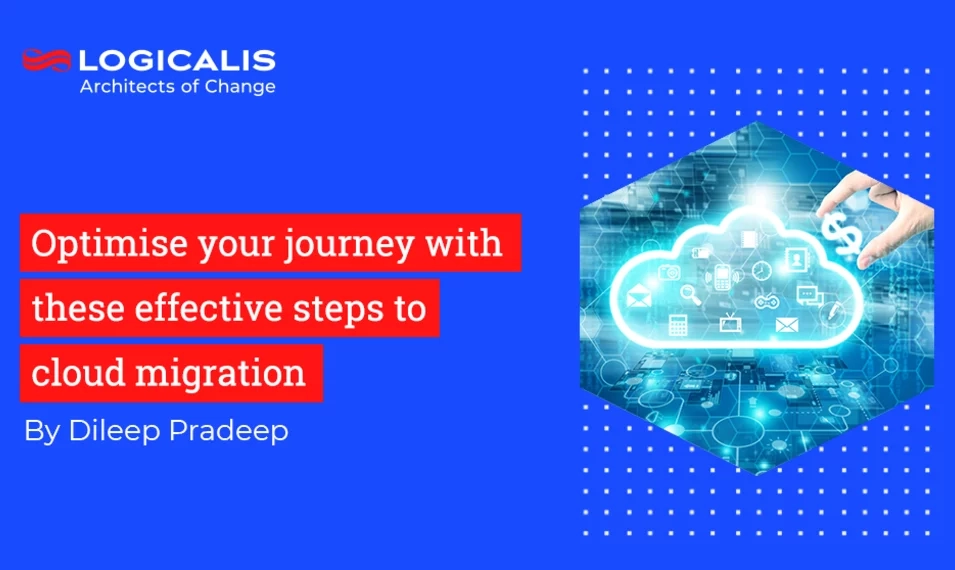
Australia , Apr 26, 2024
Optimise your journey with these effective steps to cloud migration
Cloud computing is fast becoming critical for organisations that need to work from anywhere, optimise costs, scale the business up or down, or empower company-wide innovation.
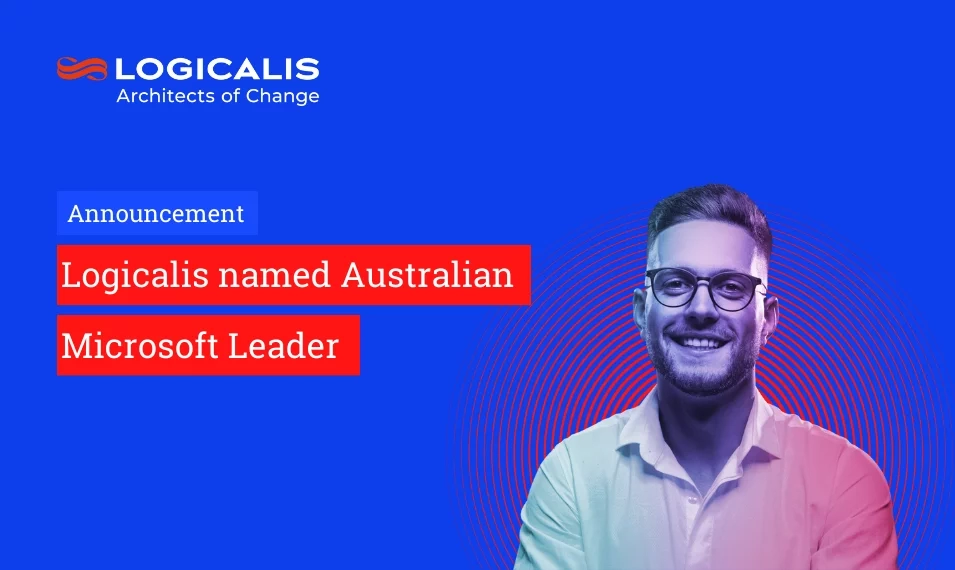
Australia , Apr 15, 2024
Logicalis named Australian Microsoft Leader
Logicalis has been named leader in two quadrants of the ISG Microsoft ecosystem report.
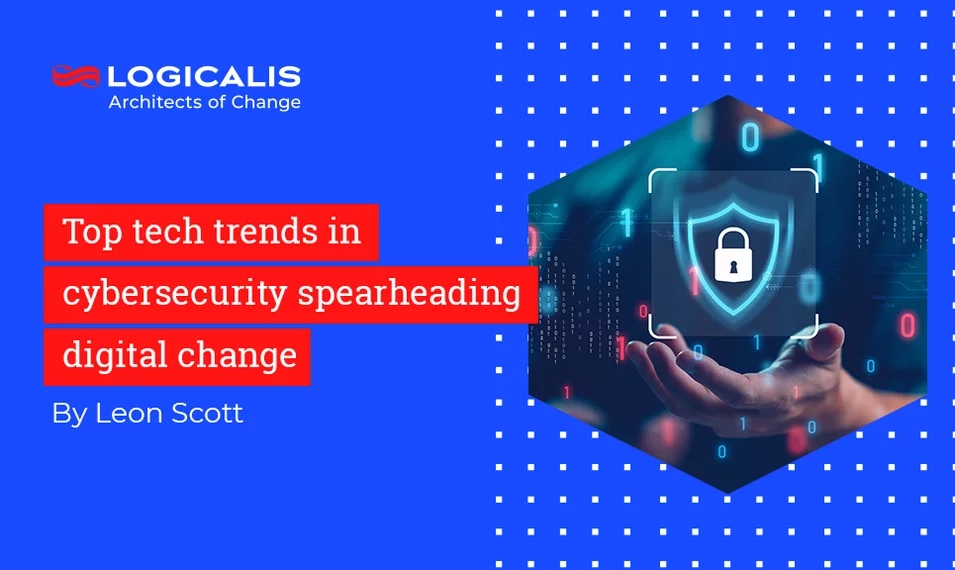
Australia , Apr 15, 2024
Top tech trends in cybersecurity spearheading digital change
Cybersecurity is an essential consideration for any organisation undergoing digital transformation. While the focus on cybersecurity in Australia has undoubtedly increased due to rising cybercrime and several high-profile attacks, current trends are causing businesses to rethink their approach.
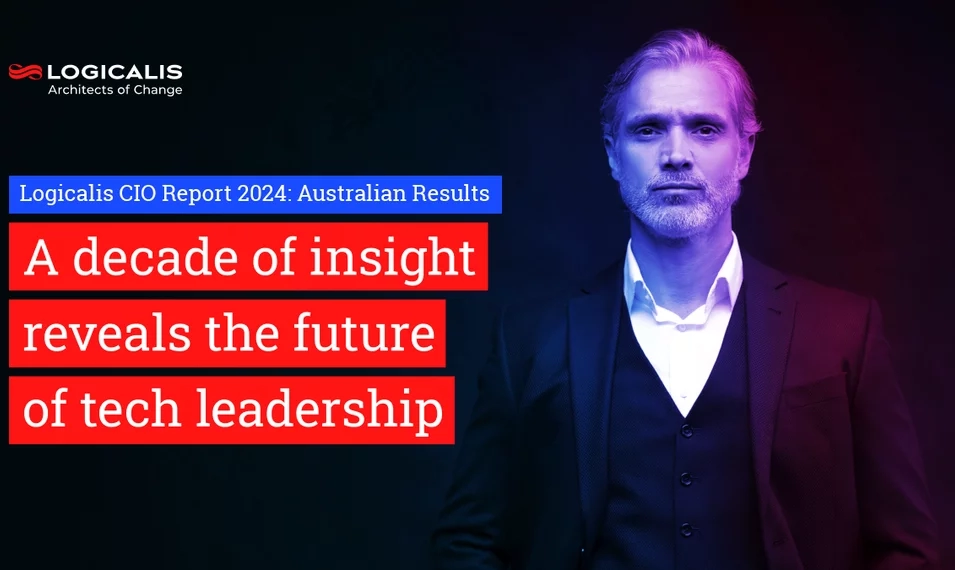
Australia , Apr 1, 2024
A decade of insight reveals the future of tech leadership in the Logicalis Global CIO Report 2024
In the ever-changing landscape of tech leadership, we are pleased to deliver the first Australian CIO Report, offering a localised perspective on the global findings.
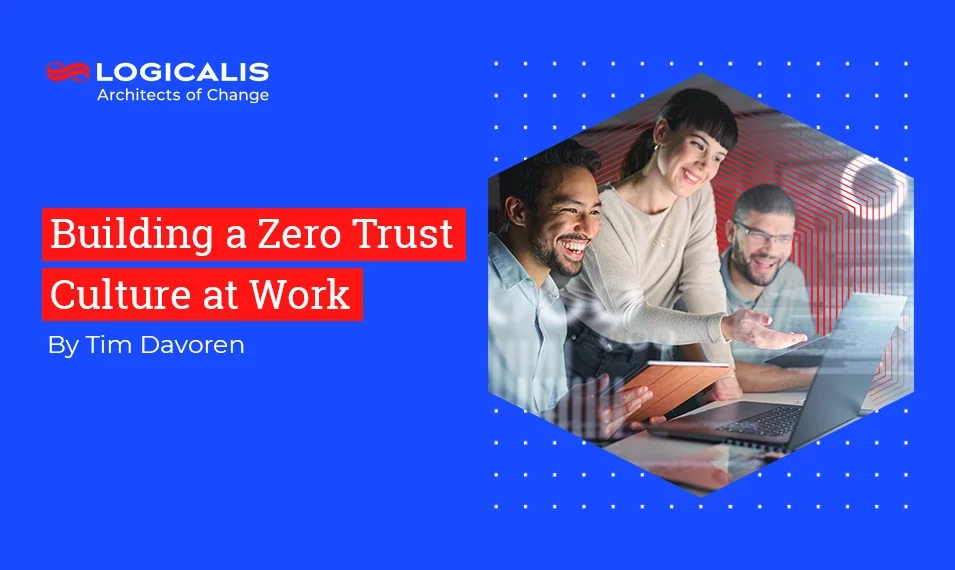
Australia , Mar 26, 2024
Building a Zero Trust Culture at Work
The way we work together in modern business has changed in recent years. We're increasingly relying on collaboration and co-delivery with partner organisations to achieve business goals and remain competitive.
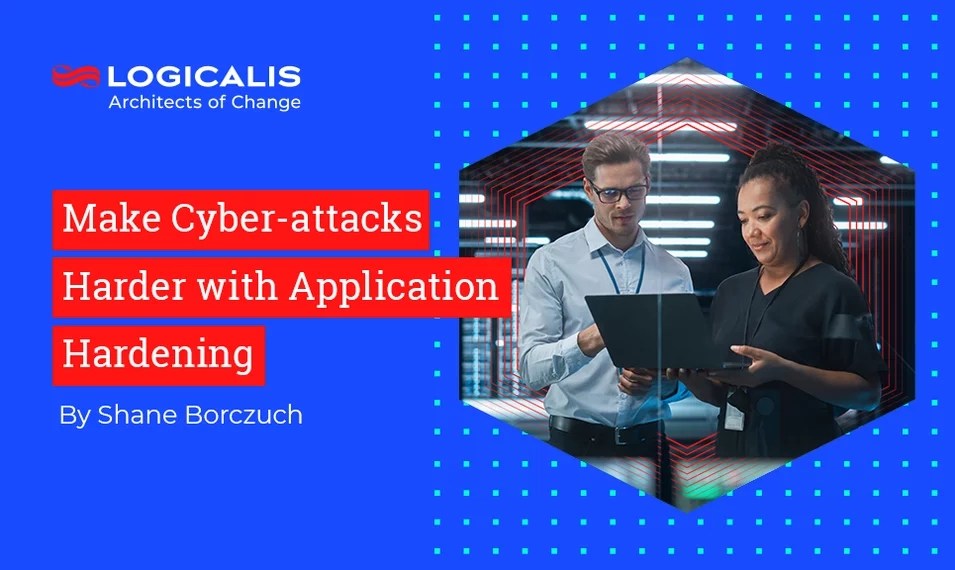
Australia , Mar 7, 2024
Make Cyber-attacks Harder with Application Hardening
For a head of IT or a Chief Security Officer, there's nothing greater than power and control, except perhaps for coming in under budget and on time for a project.
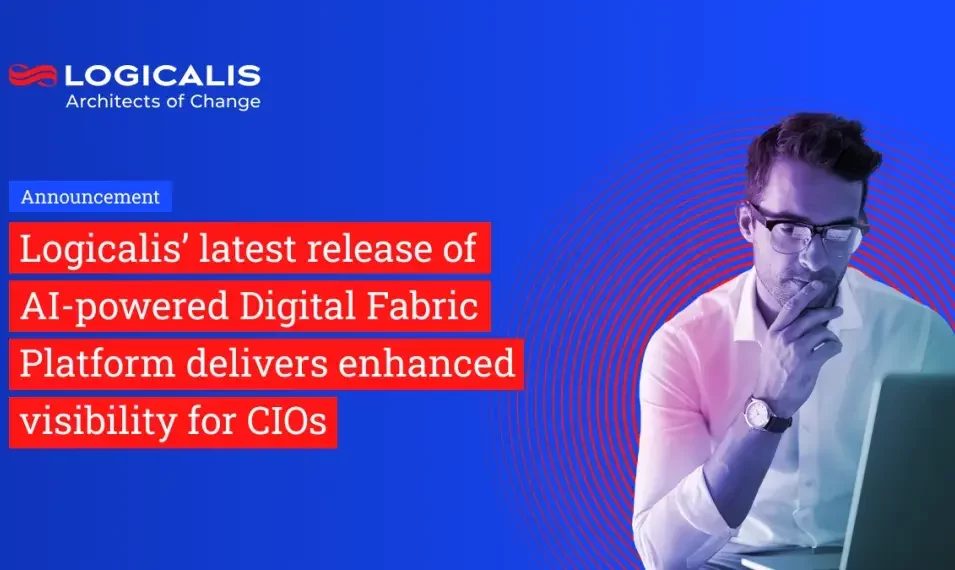
Australia , Jan 10, 2024
Logicalis’ latest release of AI-powered digital fabric platform delivers enhanced visibility for CIOs
Logicalis announces the release of the next generation of its Digital Fabric Platform, providing CIOs with deeper-level insights and recommendations to underpin the performance of their entire digital ecosystem.
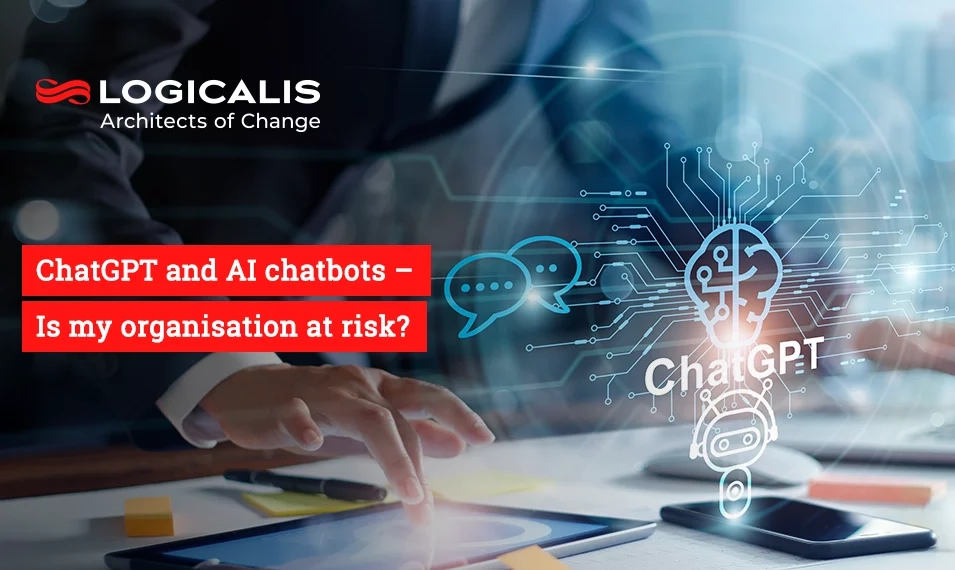
Australia , Dec 8, 2023
ChatGPT and AI chatbots – Is my organisation at risk?
Learn from our Logicalis Australia experts about how ChatGPT and AI chatbots could either enable or shake-up your business processes.
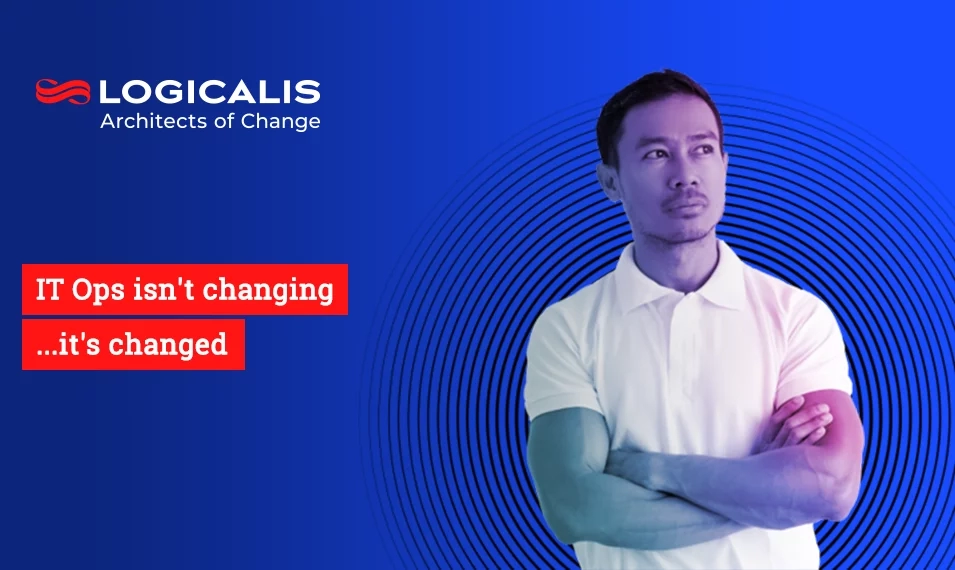
Australia , Dec 8, 2023
IT Ops isn’t changing…it’s changed
For years, the management of IT has been moving away from reactive BAU support (break-fix) and moving towards a more proactive support model. For some organisations the change is still happening; however, for the vast majority, the change has already occurred.
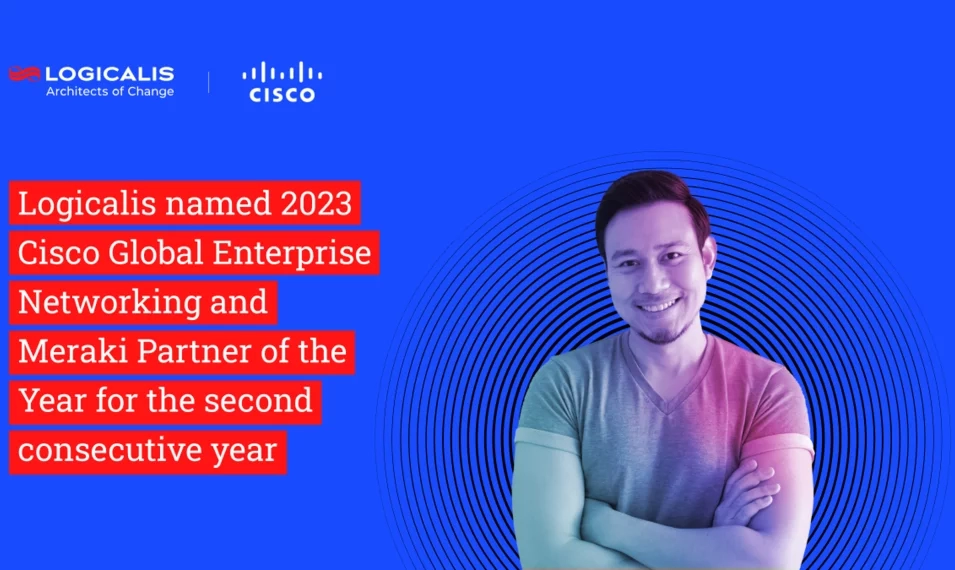
Australia , Nov 13, 2023
Logicalis named 2023 Cisco Global Enterprise Networking and Meraki Partner of the Year for the second consecutive year
Last week, we proudly received the 2023 Cisco Global Enterprise Networking and Meraki Partner of the Year award during Cisco’s annual Partner Summit. The award recognised Logicalis' global capability in Enterprise networking, with Logicalis Portugal receiving a special mention for their standout contribution to driving Intelligent Connectivity managed services to customers in Portugal.
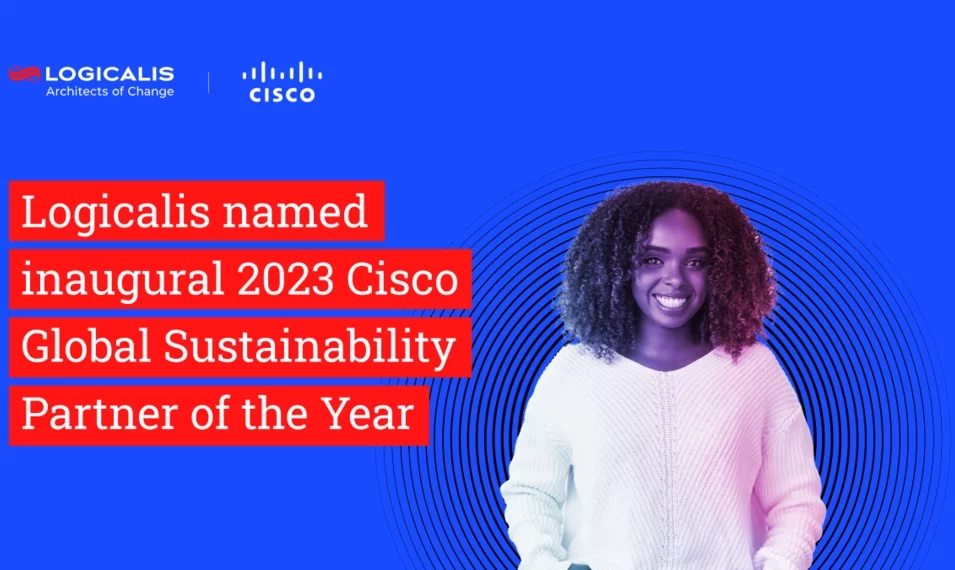
Australia , Nov 13, 2023
Logicalis named inaugural 2023 Cisco Global Sustainability Partner of the Year
Last week, we were honoured to receive the inaugural 2023 Cisco Global Sustainability Partner of the Year award during Cisco's Global Partner Summit event in Miami. This prestigious accolade acknowledges Logicalis' exceptional sustainability achievements and success in helping customers reduce the environmental impact of their digital ecosystems across the globe.
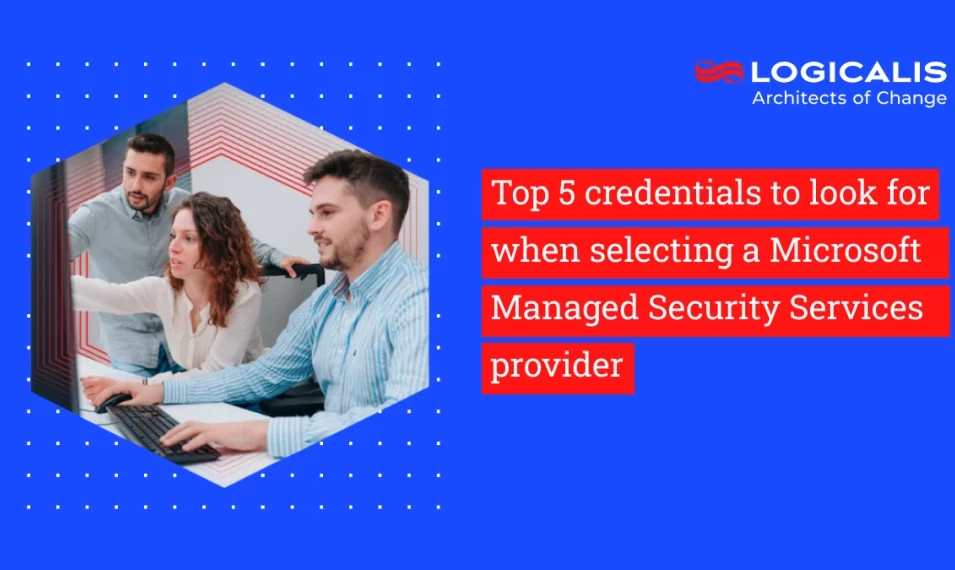
Australia , Oct 24, 2023
Choosing the right MSSP -Top 5 credentials to look for when selecting a Microsoft Managed Security Services provider (MSSP)
Our recent CIO survey shows over half of respondents plan to increase their risk management investment. They also consider malware and ransomware significant risks that their organisations will face in the coming year.
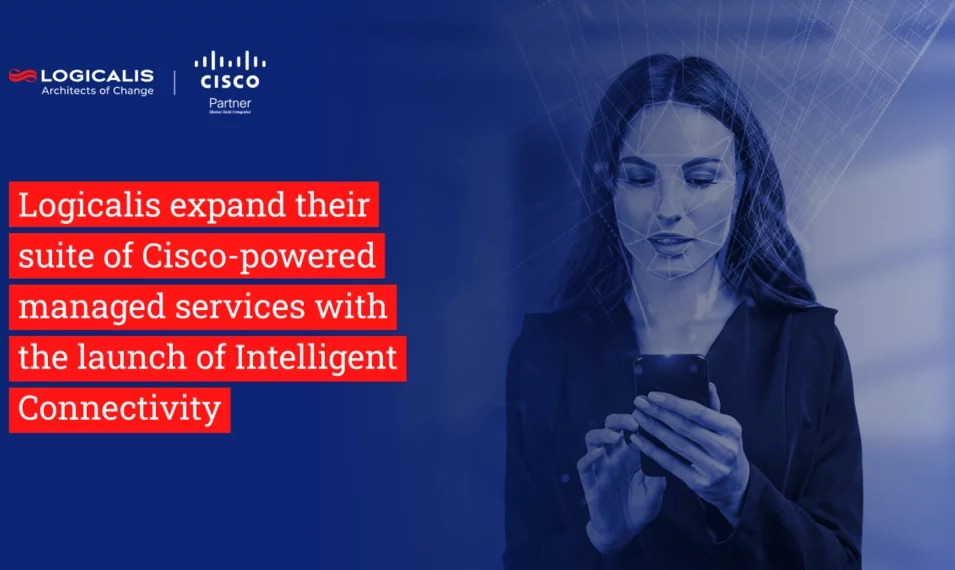
Australia , Sep 21, 2023
The power of next generation connectivity
To be able to succeed, leaders need to create environments that can adapt to maximise opportunities, mitigate risks and most importantly be able to scale both securely and sustainably. The key to this is connectivity.
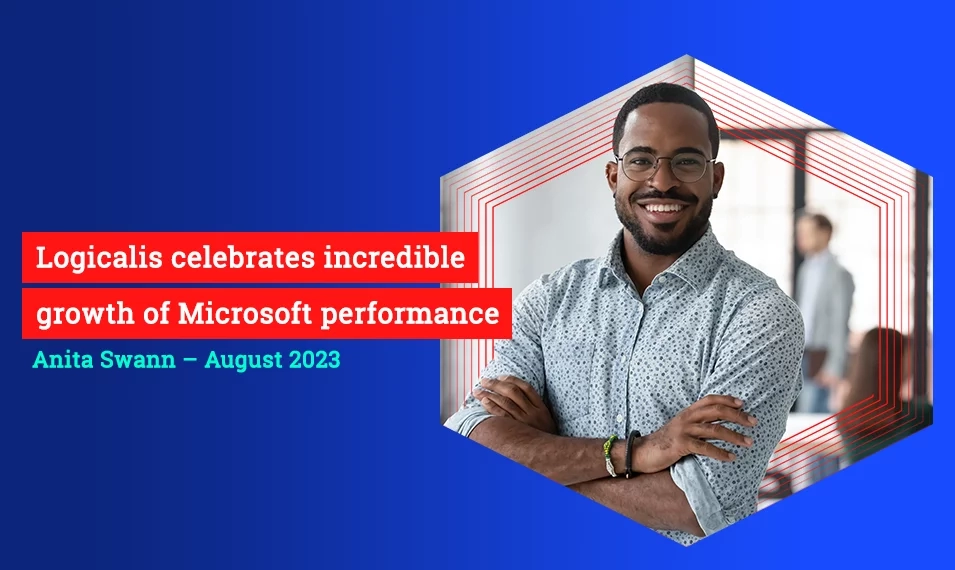
Australia , Aug 9, 2023
Logicalis celebrates incredible growth of Microsoft performance
FY23 has been an extraordinary year for Logicalis’s Microsoft partnership. We’ve seen 60% year-on-year growth reflecting our unwavering commitment to helping customers deliver business value through digital transformation.
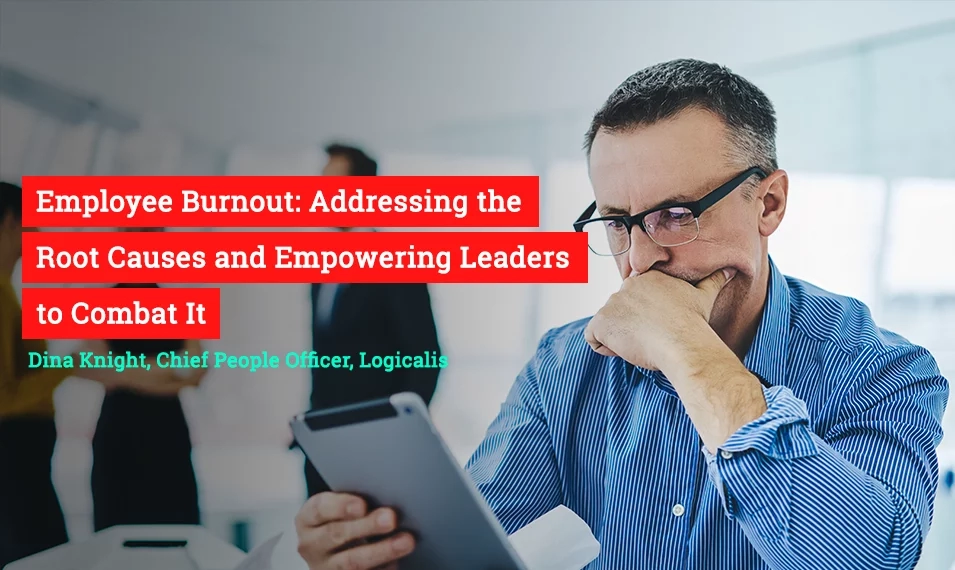
Australia , Jul 10, 2023
Employee Burnout: Addressing the Root Causes and Empowering Leaders to Combat It
Employee burnout has become an increasingly alarming concern in recent years, and the COVID-19 pandemic has only exacerbated the issue. With the pandemic forcing many employees to work remotely, isolation and blurred boundaries between work and home life have led to a significant increase in burnout cases.
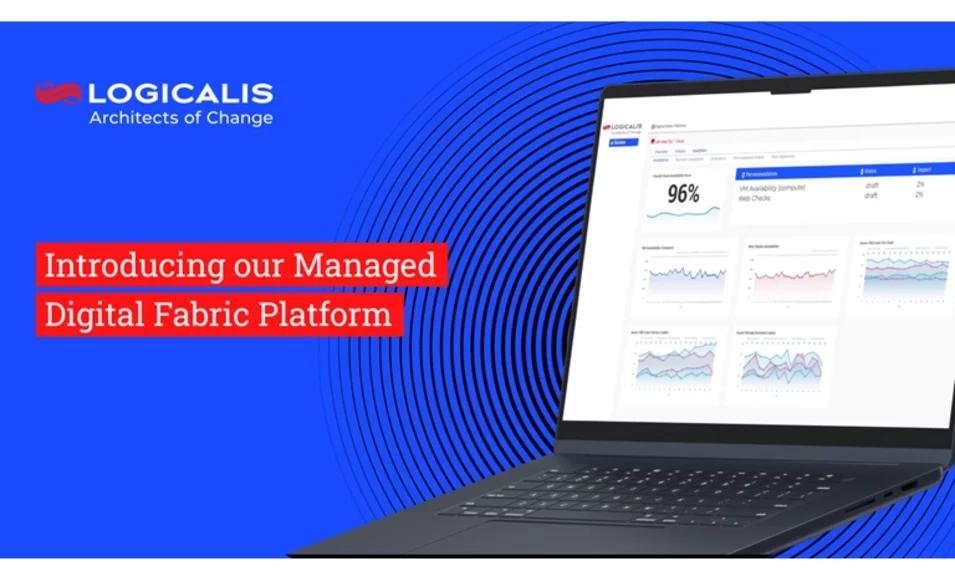
Australia , May 12, 2023
Introducing our Managed Digital Fabric Platform
Hear from Logicalis CTO, Toby Alcock, as he describes the challenges customers are facing and how our Digital Fabric Platformm can enable them to drive business outcomes.